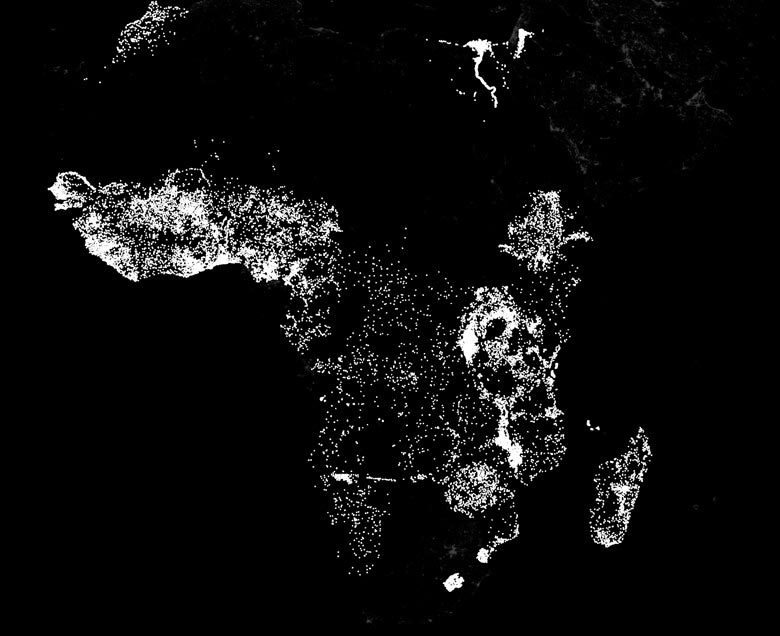
Mapping Poverty Using Machine Learning And Satellite Imagery
The way we map the world is changing fast. One of the most detailed population density maps of the world was created by Facebook, a company that until recently no one would have associated with maps, of any kind (Read: Facebook created the most detailed Population Density Map to bring the rest of the world online). We are beginning to usher in a new era in mapping – an era in which maps generated by machine learning algorithms.
Machine Learning and Satellite Imagery
By 2030, United Nations hopes to eradicate poverty worldwide and in the data-driven world that we live in, its extremely important to measure our progress towards that goal. The traditional way to measure progress would be to undertake ground surveys. A team from Stanford university has another method to map poverty, using deep learning and satellite imagery.
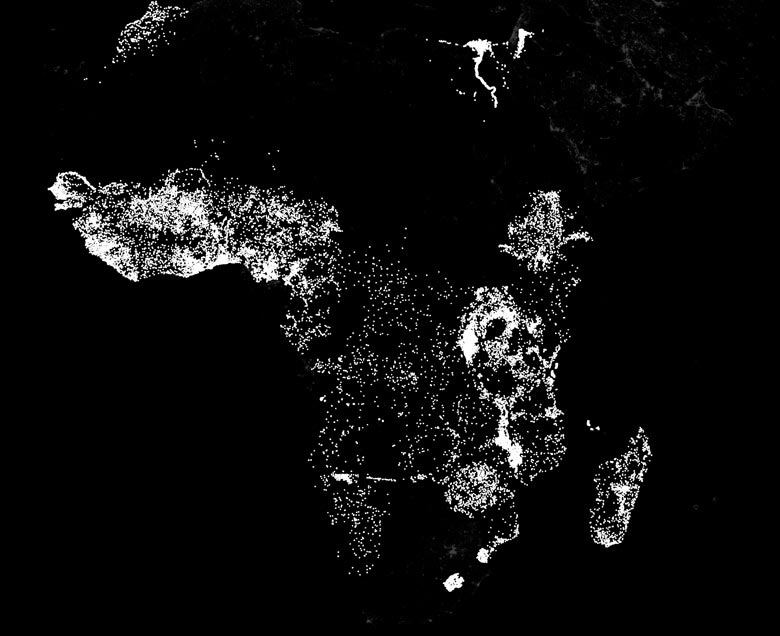
Stanford researchers use machine learning to compare the nighttime lights in Africa – indicative of electricity and economic activity – with daytime satellite images of roads, urban areas, bodies of water and farmland. (Image from Stanford.edu)
In the paper titled “Transfer Learning from Deep Features for Remote Sensing and Poverty Mapping“, the interdisciplinary team comprising of researchers from the Computer Science and Earth System Science department have introduced a new transfer learning approach for poverty estimation based on satellite imagery.
Mapping Poverty Using Satellite Imagery
Electricity and nighttime lights are one of the modern indicators of economic activity and the lack of artificial lights during night time is regarded as a sign of relative poverty and a machine learning system that has access to a treasure trove of day time and night time satellite imagery together with “ground truth” (poverty data collected using traditional methods) can come up with some interesting associations which can be then used to map poverty in areas where such ground truth.
“Basically, we provided the machine-learning system with daytime and nighttime satellite imagery and asked it to make predictions on poverty,” said Stefano Ermon, assistant professor of computer science. “The system essentially learned how to solve the problem by comparing those two sets of images.” – Stanford.edu
The research work has been nominated for NVIDIA’s 2016 Global Impact Award (Read: How GPUs are helping Map Worldwide Poverty). If you are interested in learning more about the work – check out the post on Stanford University’s page.
What Next?
Facebook already proved how useful machine learning can be to extend the capabilities of remote sensing. Training the computer to classify an area based on training samples is a technique that has been applied in image processing since a long time now but the algorithms where always computational expensive and didn’t scale well enough to be applied on a global scale. Machine learning and deep learning are providing a means to scale it to a global level, taking remote sensing to ever greater heights.
Even as they consider what they might be able to do with more abundant satellite imagery, the Stanford researchers are contemplating what they could do with different raw data – say, mobile phone activity. Mobile phone networks have exploded across the developing world, said Burke, and he can envision ways to apply machine-learning systems to identify a wide variety of prosperity indicators.
“We won’t know until we try,” Lobell said. “The beauty of machine learning in general is that it’s very useful at finding that one thing in a million that works. Machines are quite good at that.” – Stanford.edu
Did you like this post about mapping poverty with AI? Read more and subscribe to our monthly newsletter!
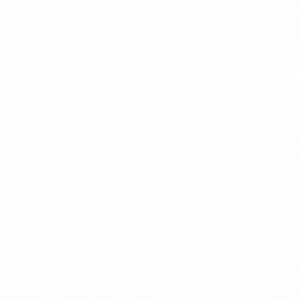