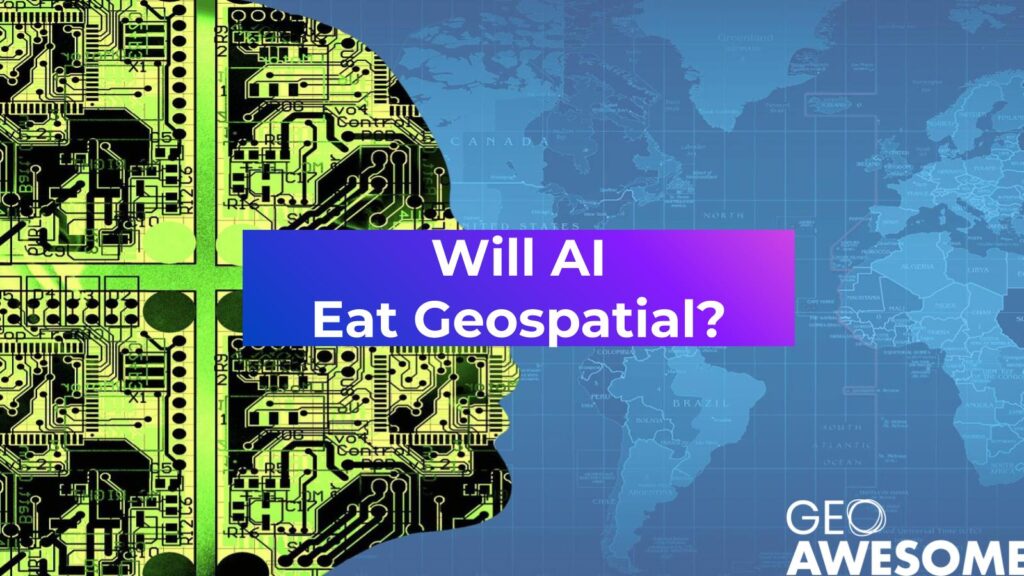
Will AI Eat Geospatial?
The LinkedIn post by Will Cadell and the question raised by Peter Rabley during his keynote at GeoIgnite 2025 were just too catchy to ignore, even though I wasn’t in the room to hear the keynote live. The question isn’t just catchy because it taps into general concerns about AI, it also challenges a long-held belief in the industry: “spatial is special.” It surfaces a deeper tension within the geospatial community of practitioners (as Will called them in one of his recent blog posts)
are we building the future, or is the future being built around us?
So will AI eat geospatial? …Not Exactly, But It Will Redesign the Menu
AI isn’t exactly going to replace geospatial and but it is certainly going to make it way more easier to abstract some of the unneccesary complexity that limits the adoption of the technology. AI is already changing workflows, expectations and architectures in ways we cannot ignore.
As a grad student in the late 2000s, one of the most boring things that I had to do was to manually digitalise several datasets including bathymetry maps to then carry out a GIS analysis to identify where polymetallic nodules might be found. My dad jokingly called it “geospatial painting” because all I seemed to be doing was tracing lines on a screen (and yes, it was exactly what I was doing). It’s hard to imagine that kind of manual work being necessary today. AI can now extract and digitize contour lines from scanned maps in minutes.
AI is fundamentally shifting how geospatial tasks are approached. Imagery analysis, which once relied heavily on manual classification, is now being handled by deep learning and foundation models capable of interpreting complex patterns. Traditional data fusion methods, typically based on stacking layers within a GIS, are giving way to AI-driven techniques that can integrate diverse sources such as SAR, optical imagery, vector data, and IoT feeds in a more dynamic and contextual manner. Searching spatial data no longer depends solely on coordinate-based queries or bounding boxes; natural language interfaces are emerging, allowing users to ask location-based questions conversationally. Cartography is also evolving, static maps are being replaced by generative, context-aware representations that can adapt based on user needs. Finally, change detection, previously guided by fixed thresholds, is increasingly managed by AI models trained to identify anomalies over time. These shifts point to a broader transformation: from geospatial tools to geospatial reasoning.
This shift demands more than new algorithms. It requires rethinking the structure of spatial data itself. One notable recent effort is the OGC’s AI+DGGS Pilot, which reimagines geospatial architecture to align with the strengths of AI. Using Discrete Global Grid Systems (DGGS), the project lays the groundwork for scalable, consistent, and AI-ready data systems. It is not just about integrating AI into GIS; it is about making geospatial data interoperable, structured, and ready for reasoning at scale.
So yes, AI will consume
-
Repetitive workflows
-
Manual image labeling and feature extraction
-
Rigid pipelines that cannot scale or adapt
-
Low-value services that offer little beyond automation
We still need to figure out the core essence of the work: driving insights from the data by asking better questions and helping decision-makers make more informed decisions.
What Powers AI? Magic? Nope it’s Data!
As Andrew Ng, co-founder of Google Brain and Coursera, put it:
“The dominant paradigm over the last decade was to download the dataset while you focus on improving the code. But for many practical applications, it’s now more productive to hold the neural network architecture fixed, and instead find ways to improve the data.”
— Andrew Ng, Data-Centric AI, NeurIPS 2021
Great Geo+AI does not start with a model. It starts with thoughtful, well-structured data and the geospatial expertise to get it right. Which makes good quality labeled data to train your AI application even more important. No wonder that we have a rise in the number of companies specializing and providing high-quality labeled geospatial data.
A recent guide by Kili Technology offers a practical reminder. Earth Observation data is noisy, heterogeneous, and challenging to annotate. Whether identifying rooftops, detecting roads, or classifying land use, the performance of AI models is directly tied to the quality of labeled data they are trained on.
The guide makes it clear that AI does not eliminate the need for human input. It increases the demand for domain expertise, structured workflows, and quality control. Looks like the human in the loop isn’t exactly yet a thing of the past. In this context, AI is not eating geospatial. It is relying on (geospatial) professionals to feed it high-quality, annotated, and context-rich data.
Reality Check: Trust, Ethics, and Governance
As Anusuya rightly points out in her post on the topic, AI has been part of the geospatial for a while now but now time to innovate, govern and collaborate better. She has wonderfully summarized the key points in her reflections:
-
Performance of AI trained on synthetic data is degrading
-
Customer trust in AI-generated content is declining
-
AI-related incidents rose by 56 percent last year
These are not minor issues. They signal deeper challenges in the scalability and governance of AI systems. Peter’s call to action was clear. Moving forward, the geospatial sector must:
-
Embed ethics and human oversight from the start
-
Prioritize data integrity, provenance, and trust
-
Embrace openness and minimize environmental impact
These principles are enablers of innovation.
Its time to evolve
So, will AI eat geospatial?
No. But it will reshape the value chain. And it will expose which parts of our field are ready to evolve and which are overdue for reinvention. AI is not coming for maps. It is coming for process inefficiencies, siloed data, and workflows that cannot scale. That is an opportunity.
The real work now is not to defend geospatial from AI. It is to redefine what geospatial can become with AI. Better tools, better decisions, and better systems for the world we are mapping and managing.
Let us not just respond to the future. Let us design it.
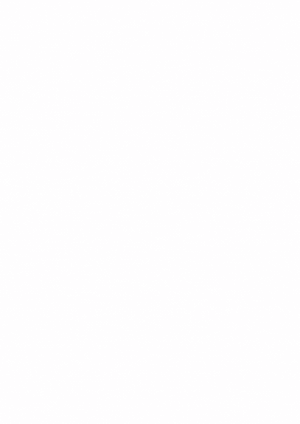
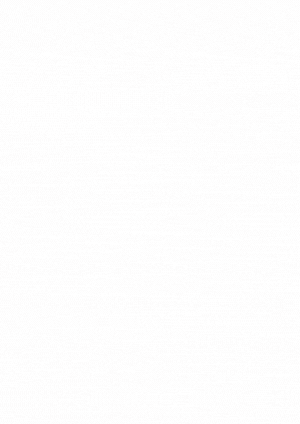