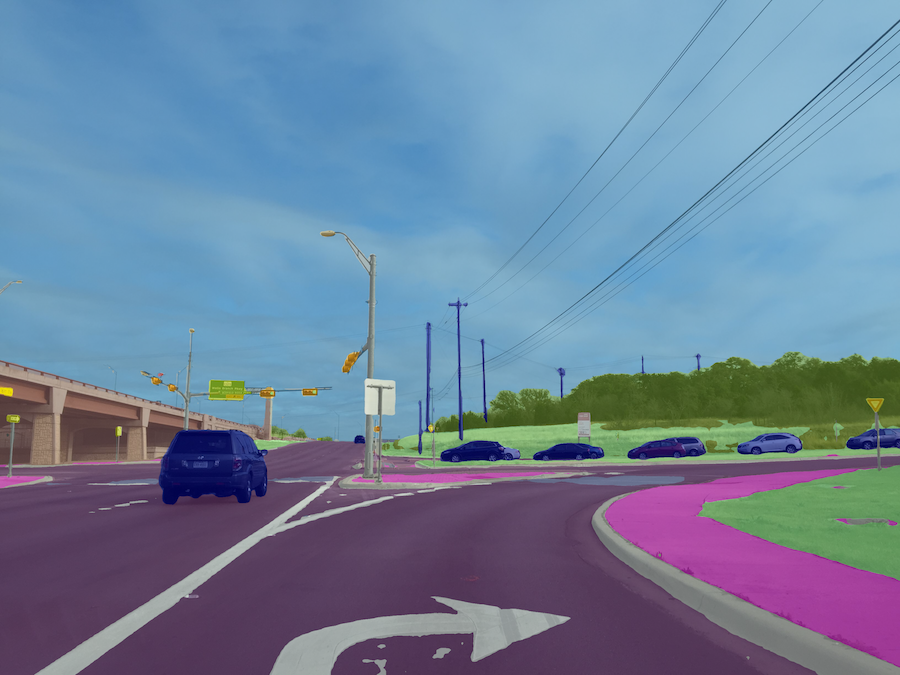
Mapping and Machines: Level up with AI
In a recent article, former Apple Maps designer Justin O’Beirne showed the stunning increase in coverage and detail of building outlines on Google Maps, thanks to using computer vision techniques. By extracting building outlines from satellite imagery together with place and business information from street view imagery, Google generated a new set of data on “areas of interest” to display on their maps—something that takes months of work for humans to do for just one city.
Computer vision helps us harness machines to understand the world. There are millions of details around us as we walk down a street, and a human is able to understand them fairly easily. For the buildings lining the street, we know how many levels they have and whether they’re businesses, residential buildings, schools etc. We also understand the vehicles and know what to expect from their behavior.
Teaching machines to “see” is no simple task. The computer interprets an image by assigning every pixel into a category—formally called semantic segmentation. Clusters of similarly labeled pixels form segments of the image, each representing a different real-world object or feature.
This is how a well-taught machine sees the street scene (different objects are color coded)
Once trained, the computer is able to process, and perhaps even more importantly, output this information immensely faster and on a larger scale than humans ever could.
While machines will be able to do the bulk of the work, they won’t be able to do it completely on their own. Humans are needed to supervise—first, to train the algorithms, then to check up on the results and step in to correct the toughest cases. This is what is meant by the Human in the Loop approach.
When it comes to mapping, to understand the contents of an image is just one half of the process. The other half is to figure out the geographic location of the objects detected. Again, the machine works this out similarly to a human.
As humans, we need stereo vision in order to understand an object’s position in space. Each of our eyes sees the same object from a slightly different angle; in other words, each eye captures a separate image where the same object is visible. That’s what we also need for computer vision: record the same object in at least two images in order to triangulate its position and reconstruct it in 3D space. The difference again is that a computer can do this so much faster and more accurate.
3D reconstruction of a street in Amsterdam based on street-level images
Imagery is collected not only by map companies but also cities, local governments, road authorities, individuals, and many more. Pooling them together and processing this with computer vision means that maps can get updated much faster than before, keeping up with the requirements of freshness, coverage, accuracy, and high level of detail. As my colleague Chris also recently discussed, machines won’t be the only source of geospatial data—but to let them do the heavy lifting is the only scalable way. Human labor will be much better used when invested into verifying and adjusting the details and training the machines.
Referring to O’Beirne once more, he makes a note that “It’s interesting to ponder what this means for Google’s competitors. It’s no longer enough to simply collect data. Now to compete with Google, you also have to process that data and make new features out of it.” And he raises the question of what this means for OpenStreetMap.
I’m convinced it means something amazing. Imagery processed with computer vision is already available to OpenStreetMappers. The gap between Google and OpenStreetMap is still fairly large but more and more company backers are putting resources into OpenStreetMap (for example Facebook and Mapillary). Combined with a motivated and hard-working community, there’s a good chance that they’ll be able to make maps that can shake today’s mapping giants.
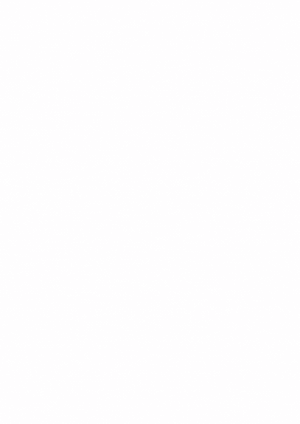
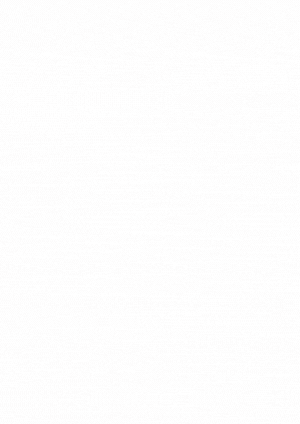