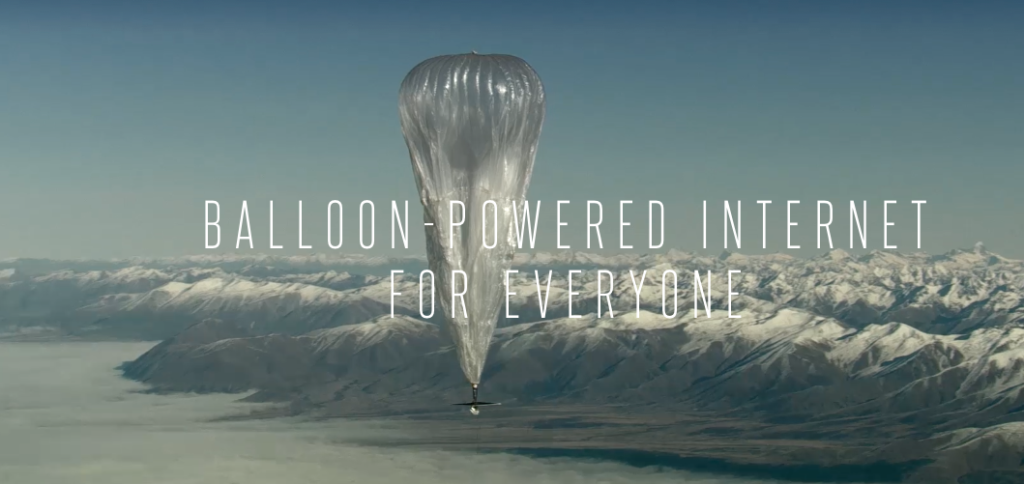
How Machine Learning is helping Google to enhance wind prediction
When Google first launched its moonshot “Project Loon” to provide internet connectivity to farthest corners of the world by launching dozens of balloons, the thought process was, as one balloon drifted away, another would be ready to take its place. A continuous stream of balloons was to circumnavigate the world on a daily basis. The project which was unofficially started back in 2011 is now much closer to reality than it ever was, all thanks to – Machine Learning!
Machine Learning for wind prediction
One of the biggest hurdles for the success of Project Loon was the number of balloons Google would have had to launch if they were to maintain internet connectivity over a particular location. Over time, the team behind Project Loon figured that with updates to their navigation technology, they were able to maximize the time a balloon spend over areas internet connectivity was required.
“We wondered, what if instead of circling the world, we could ride these winds in small enough loops to cluster balloons over a single area? Forget a ring around the world – just hang out!” – Project Loon – Improving Navigation
At 20 Kilometers above the Earth’s surface in the Stratosphere where the Ballons are traveling, the winds are stratified with each layer of wind varying in velocity. Google’s team began using predictive models of the winds and decision-making algorithms to move each balloon up or down into a layer of wind blowing in the right direction, to get the Ballons to go where they wanted them to go. Last summer, they put those updates to the test in Peru, managing to keep the balloons drifting within Peruvian airspace for a total of 98 days!
It’s amazing to see how Google’s engineers were able to use Machine Learning algorithms to predict wind patterns with such accuracy. Many weather researchers would love to get their hands on the data and algorithm. Apparently, the navigation system onboard the balloons were able to predict the wind patterns using relatively small amounts of data using an artificial intelligence technique known as Gaussian Processes. (Related: AI Is About to Learn More Like Humans—with a Little Uncertainty)
For now, most of what machine learning can accomplish takes place in the purely digital realm. But as the Project Loon experiment shows, these systems have the potential to play a role not only online but in the physical world, too. And not just with driverless cars. – Wired
It’s true that Machine Learning has largely been used to analyze phenomena that are purely in the digital realm but perhaps the biggest benefits of using Machine Learning will be in the physical world. It will not be long before Machine Learning algorithms are able to predict the weather better than our current systems, helping us farm better and help feed the 7 billion people that call the Earth their home.
Did you like this article about ML and wind prediction? Read more and subscribe to our monthly newsletter!
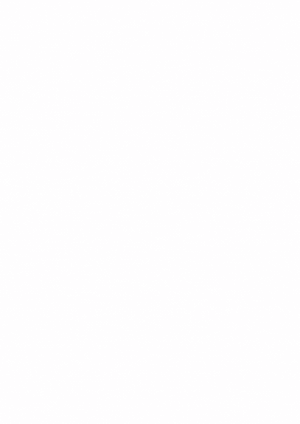
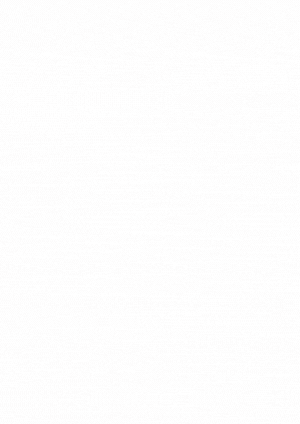