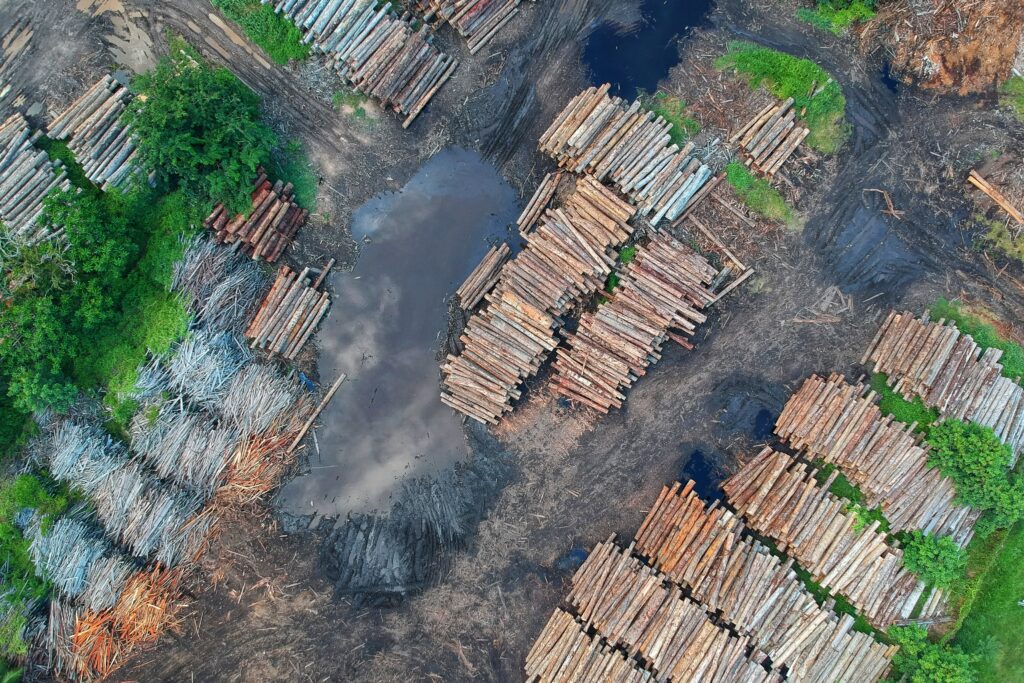
Why AI is the Key to Stopping Global Deforestation
Editor’s note: This article written by the Satelligence team is part of the annual GeoTrends series. GeoTrends series aims to provide a platform for thought leaders, executives and strategic thinkers in the industry to articulate their vision and help our readers understand where the industry is headed in the future.
AI is proving to be the key to unlocking the supply chain quagmire; empowering farmers at the frontline of supply chains to lead the fight against deforestation by making sure they’re fairly compensated.
Deforestation related to agriculture is responsible for 15% of global greenhouse gas emissions.
A wave of sustainability commitments from some of the world’s largest businesses has created unprecedented demand for tools that can help companies identify and mitigate deforestation in their supply chains. New laws such as the EU’s Regulation on Deforestation Free Products will soon place mandatory obligations on companies to eliminate deforestation from their supply chains in order to keep trading on the EU market.
In order to stay within our planetary limits, companies and countries know they must act fast to make deforestation-free supply chains a reality. But company supply chains are huge operations spanning multiple countries, companies, and legal frameworks.
How AI Empowers Farmers To Fight Climate Change
On the one hand, smallholder farmers in the first mile of supply chains are squeezed from both sides by a need to clear more land for expansion and by demands for emissions reductions from manufacturers.
On the other, the race to net zero has corporations around the globe cutting emissions to meet climate commitments. For now, some of these emissions are unavoidable, so companies are compensating with carbon offsets.
For the first time, developments in AI machine learning have made it possible to measure precisely how much carbon has been captured and sequestered as a result of the agroforestry practices on individual smallholder farms. The carbon is converted into CRU’s (Carbon Removal Units) which are sold on the Voluntary Carbon Market. As much as 80% of the revenue from the sale can go directly to farmers, providing a significant incentive to nurture the trees on their land rather than cut them down.
CRUs are ex-post, meaning that each Unit sold represents one tonne of carbon that has already been removed from the atmosphere. This differs from the majority of carbon credits, which are sold before removal. More than just a novel application of AI, this nuance helps assuage concerns over the speculative nature of the carbon market.
Fulfilling the Potential of Applied AI: How does it work?
An AI-powered carbon estimation machine learning model based on satellite imagery is used to estimate the amount of stored carbon in plots at regular intervals. Those plots represent farm plot boundaries, which are catalogued in partnership with industry and local NGOs.
In order for machine learning to predict carbon stock, three components are needed:
- Farm plot field data as a training set
- Satellite imagery as a feature set
- A Random Forest machine learning model
With these ingredients, you can teach the model to estimate carbon levels based on the feature set to track changes over time. It can convert satellite images of plots into units of sequestered carbon that are organised farm by farm.
Improving Accuracy Over Time
Scalability is crucial, and repeatability and traceability are the cornerstones of scalability. One of the biggest challenges in using AI to achieve scalability is coping with variations in farm plot field data, as different companies across different countries submit data in different ways. Divergent interpretations and a lack of homogeneity across training data sets create issues with local bias. The predictive capability of the model can only ever be as good as the training data set it’s drawn from.
In practice, this means that modelling approaches are tied to the ecological region they’re drawn from. They aren’t transferable to locations where the environmental conditions are entirely different.
AI machine learning has been crucial in overcoming these obstacles. an ISAE-3000 Type 1 Certified quality control audit process has been developed for each type of environment, country or commodity, a form of meta-algorithm, that allows carbon modelling approaches to be adapted to any ecological region.
With the ISAE-3000 certified algorithm, companies can use the same quality control process to map Cocoa in the Ivory Coast as they could to Palm oil in Indonesia.
AI Facilitates Human Creativity
The project leverages the ability of AI to discover and repeat patterns that are too complicated for humans to recognise, or too boring for them to repeat, enabling engineers to apply their expertise to analyse, curate and fix errors in the data. Experts are then free to focus on fixing external abnormalities like clouds, atmospheric disturbances and noise, so the product can be continuously improved.
Thanks to innovations in AI, the otherwise costly and complex process of scaling carbon footprinting measurements across global commodities is getting simpler by the day.
Did you like the article? Read more and subscribe to our monthly newsletter!
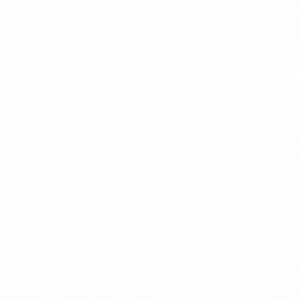