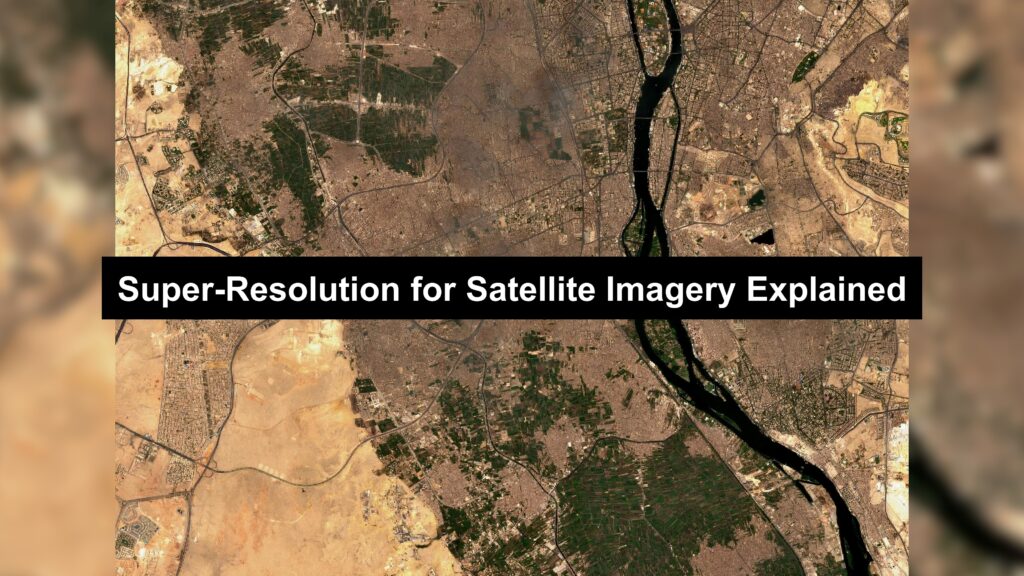
Super-Resolution for Satellite Imagery Explained
The advent of satellite imagery has revolutionized our ability to monitor and understand the Earth’s surface. From tracking environmental changes to aiding in urban planning, satellite imagery provides invaluable data.
However, the resolution of these images often limits their utility. This is where super-resolution technology comes into play, offering the potential to enhance the clarity and detail of satellite images.
This article explores the technological advancements in super-resolution, its applications across various industries, and a case study demonstrating its efficacy.
Technological Advancements in Super-Resolution
Super-resolution (SR) refers to a set of techniques aimed at increasing the resolution of images. These methods can be broadly categorized into optical and geometrical or computational super-resolution.
- Optical super-resolution techniques enhance the resolution beyond the inherent optical limitations. So for satellite imagery, this involves hardware-based approaches such as advanced optical systems that can capture higher resolution images directly from space.
- Geometrical super-resolution, also known as computational super-resolution, uses image processing algorithms to enhance the resolution of digital images after they have been captured. In satellite imagery, this involves processing low-resolution images to create higher-resolution outputs.
Geometrical/computational super-resolution is particularly useful for satellite imagery as it allows for the enhancement of existing images without requiring changes to satellite hardware.
Traditional interpolation methods, such as bilinear or bicubic interpolation, often fail to provide significant improvements in detail. Recent advancements leverage machine learning and deep learning to achieve superior results.
Key innovations include:
Deep Learning Models for Super-Resolution
One prominent approach involves the use of Convolutional Neural Networks (CNNs). The SRCNN (Super-Resolution Convolutional Neural Network) is a pioneering model that has demonstrated significant improvements over traditional methods. More advanced models, such as Generative Adversarial Networks (GANs), specifically the Enhanced Super-Resolution GAN (ESRGAN), have pushed the boundaries further by generating highly realistic high-resolution images from low-resolution inputs.
TensorFlow Hub and Satlas-Super-Resolution
Platforms like TensorFlow Hub provide accessible models for image enhancement, including super-resolution. For instance, the tutorial illustrates how to use pre-trained models to enhance image resolution effectively. Additionally, projects like Satlas-Super-Resolution offer specialized models tailored for satellite imagery, addressing the unique challenges posed by this domain.
Transforming Industries: The Impact of Super-Resolution
Super-resolution has profound implications across various industries, enhancing the utility of satellite imagery in numerous ways. By revealing details previously hidden from view, this innovation is revolutionizing decision-making processes and operational strategies in a multitude of industries:
1. Agriculture
In the agricultural sector, high-resolution satellite images are crucial for monitoring crop health, assessing soil conditions, and managing irrigation. The enhanced clarity and detail provided by these images enable farmers and agronomists to:
- Detect early signs of crop stress, disease, or pest infections
- Analyze soil moisture content and composition at a micro-level
- Optimize irrigation strategies by identifying areas of water stress or oversaturation
- Monitor crop growth patterns and predict yields with greater precision
2. Urban Planning
Urban planners rely on detailed satellite imagery to transform the way cities are designed, developed, and managed. Key use cases:
- Monitoring urban sprawl and land use changes with meter-level precision
- Identifying illegal constructions and zoning violations more effectively
- Planning green spaces and assessing urban heat islands with greater accuracy
- Optimizing infrastructure development by highlighting traffic patterns and population density at a granular level
An example is Singapore’s use of super-resolution imagery in its “Smart Nation” initiative, enabling real-time monitoring of urban development and environmental changes across the city-state.
3. Disaster Management
Disaster management is another critical area where super-resolution proves invaluable:
- Provide rapid assessments of damage in the aftermath of natural disasters such as hurricanes, earthquakes, and floods
- Enable more accurate flood mapping and prediction by detecting subtle changes in terrain and water levels
- Assist in planning evacuation and rescue routes and identifying safe zones with greater precision
- Monitor the progress of wildfires and predict their speed with enhanced accuracy
During the 2021 European floods, super-resolution satellite imagery allowed emergency responders to identify and reach isolated communities up to 24 hours faster than would have been possible with standard-resolution imagery. Copernicus Emergency Management System is one of the services that use super-resolution in flood mapping.
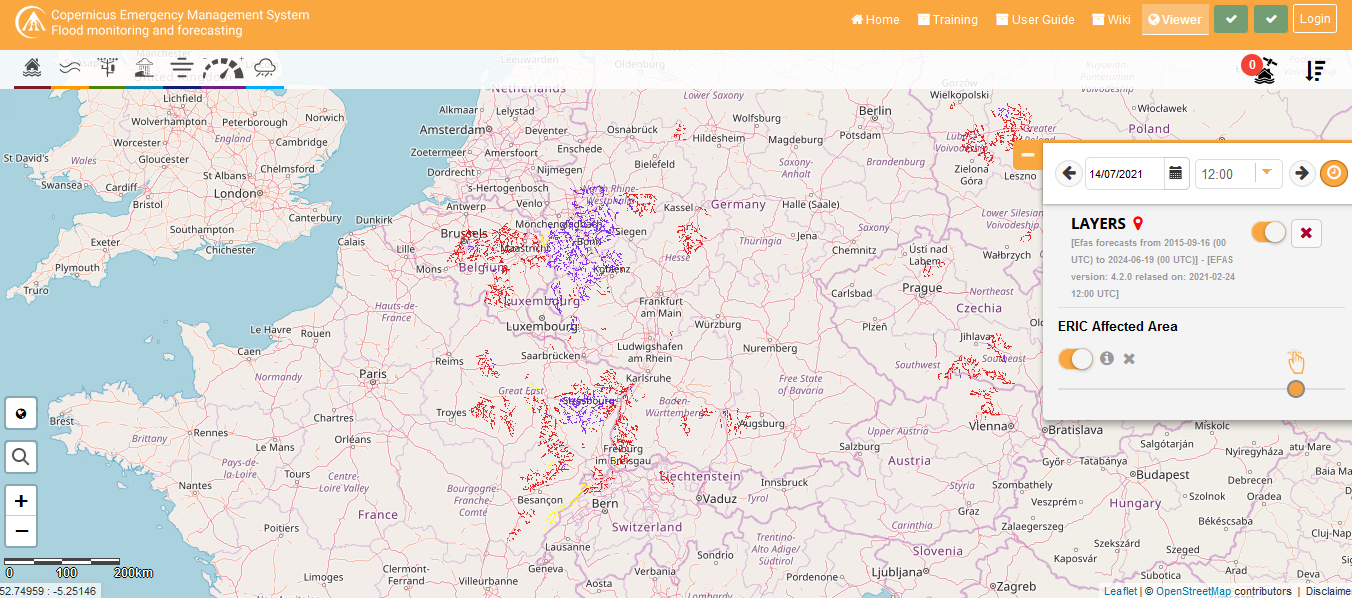
Mapping flood areas by Copernicus Emergency Management System (CEMS)
Case Study: Enhancing SentinelHub Images
To showacase the power of super-resolution in satellite imagery, we enhanced six low-resolution images from SentinelHub.
Our aim was to demonstrate how AI-driven super-resolution techniques can significantly enhance the utility and information derived from satellite data across various landscapes and urban areas.
Methodology
1. Image selection
We carefully selected six diverse images were selected representing a wide range of landscapes and urban settings:
- Urban landscapes: Budapest (Hungary) and Kraków (Poland)
- Coastal Environment: Ibiza (Spain)
- Historical Site: Giza (Egypt)
- Natural Feature: Mississippi River (USA)
- Agricultural Setting: Valensole Lavender Fields (France)
2. Processing using an AI-pretrained model
These images were initially processed using a Python script employing a super-resolution model (ESRGAN). This model was chosen for its ability to generate highly realistic textures in super-resolved images.
3. Resolution reduction
To illustrate the efficacy of super-resolution, the resolution of the original images was artificially decreased using bicubic downsampling.
4. Super-Resolution enhancement
The degrated low-resolution images were then enhanced using a super-resolution model.
Results and Implications
The results of our study were striking, demonstrating significant improvements in clarity and detail across all test images:
Urban Landscape Enhancement
- Budapest and Kraków: The super-resolution process revealed urban patterns previously indiscernible. Building outlines, road networks and even smaller urban features like parks and squares became clearly visible.
- Implication: This level of detail can revolutionize urban planning, allowing for more precise infrastructure assessment and development strategies.
Coastal and Water Body Analysis
- Ibiza and Mississippi River: The enhanced images showed remarkable improvement in water-land boundary definition. Subtle coastal features and river meandering patterns as well as vegetation cover showed improved detail.
- Implication: This enhancement can significantly improve coastal management, flood prediction, and water resource monitoring.
Historical Site Preservation
- Giza: The super-resolution technique brought out fine details of the pyramids and surrounding structures, previously blurred in lower-resolution imagery.
- Implication: This technology can aid in archaeological studies and heritage site monitoring, allowing for non-invasive analysis of historical landmarks.
Agricultural Monitoring
- Valensole Lavender Fields: The enhanced imagery revealed individual field patterns and crop rows with unprecedented clarity.
- Implication: This level of detail can transform precision agriculture, enabling more accurate crop health assessment and yield prediction.
![]() Budapest: Original image vs. SR image |
![]() Budapest: Low-resolution image vs. SR image |
![]() Cracow: Original image vs. SR image |
![]() Cracow: Low-resolution image vs. SR image |
![]() Giza: Original image vs. SR image |
![]() Giza: Low-resolution image vs. SR image |
![]() Ibiza: Original image vs. SR image |
![]() Ibiza: Low-resolution image vs. SR image |
![]() Mississippi River: Original image vs. SR image |
![]() Mississippi: Low-resolution image vs. SR image |
![]() Valensole Fields in France. Original image vs. SR image |
![]() Valensole Fields in France. Low-resolution image vs. SR image |
The Potential for Further Enhancement
While our study demonstrates significant improvements in image quality and detail, it’s crucial to recognize that we’ve only scratched the surface of what’s possible with super-resolution technlogy.
These results could potentially be even better if the pre-trained model were further trained with additional, high-quality data. The pre-trained model used in our experiment, while highly effective, was designed for general-purpose image enhancement. However, satellite imagery presents unique challenges and characteristics that differ from typical photographic images. By further training the model with a curated dataset of high-quality satellite images, we could potentially achieve even more remarkable results
This could enhance the model’s ability to capture finer details and improve overall accuracy in distinguishing urban features, thereby providing even more valuable insights for urban analysis and planning.
Transforming Satellite Imagery for Global Progress
The application of super-resolution in satellite imagery holds massive potential for positive impact enabling global progress. Enhanced imagery can lead to better decision-making across various sectors, contributing to sustainable development, efficient resource management, and improved disaster response.
- Environmental Monitoring: High-resolution images allow for precise monitoring of environmental changes, aiding in the conservation of ecosystems and biodiversity.
- Infrastructure Development: Urban planners can design more efficient and sustainable infrastructure projects with the help of detailed satellite imagery.
- Emergency Response: During disasters, enhanced images provide critical information for timely and effective response, potentially saving lives and reducing economic losses.
- Agricultural Optimization: Farmers can use high-resolution images to optimize crop management practices, leading to increased food production and security.
Super-resolution technology represents a significant leap forward in the utility of satellite imagery. By enhancing image resolution, it opens up new possibilities for detailed analysis and decision-making across various industries.
Our case study using SentinelHub images illustrates the practical benefits of this technology, demonstrating how it can improve our understanding of the planet.
As super-resolution techniques continue to advance, their positive impact on society is bound to grow, driving innovation and improving outcomes in agriculture, urban planning, disaster management, and beyond.
For more about this topic, read our EOHub article: Enhancing Satellite Imagery Readability with Super-resolution Machine Learning Models
Did you like the article? Read more and subscribe to our monthly newsletter!