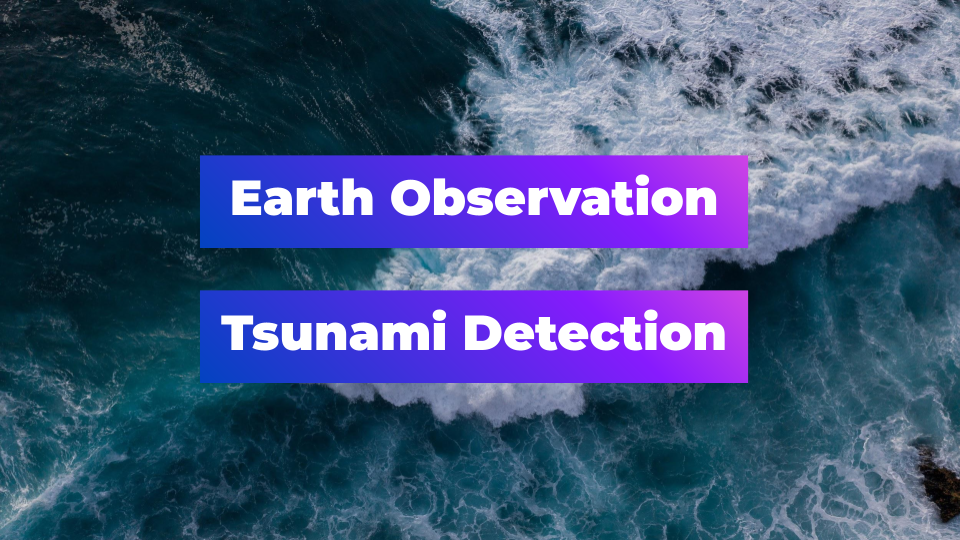
Improving Tsunami Safety with Remote Sensing Satellites
Technological advances in satellite imaging are making it possible to increasingly understand the impact of natural disasters. This is mainly due to multi-temporal satellite imagery, which is a powerful tool for disaster management and risk reduction. Multi-temporal satellite imagery combines images taken at different times, to create a more detailed picture of an area. Such images can provide valuable information on the effects of disasters such as floods, storms, earthquakes and landslides. Not every country is able to effectively deal with natural disasters, which is why the International Charter on Space and Major Disasters was established. It is a non-commercial initiative to collect and provide remote sensing data on natural disasters and catastrophes anywhere in the world to improve disaster management processes. These data are provided to organizations involved in mitigating their effects and helping those affected. The imagery is currently acquired from satellites belonging to seventeen member countries, partners involved in the Charter project, and other data providers.
A Finnish company belonging to the initiative, ICEYE, is developing a constellation of lightweight SAR satellites as part of the project, which enables monitoring, mapping and analysis of post-disaster changes detected on the Earth’s surface. The technology has been used for, among other things: assessing damage, analyzing infrastructure before and after disasters, the effects of floods, volcanic eruptions and landslides, and measuring oil spills.
Another example of satellites supporting the initiative is Planet’s PlanetScope constellation, a fleet of miniature satellites called CubeSats, which collects weekly or daily images of the entire planet, and produces about 1,700 images each month. The captured data is used to monitor the spread of wildfires, among other things. Thanks to PlanetScope’s huge data sets, 3D reconstructions or digital surface models of any area can be created.
A detailed list of all satellites providing data under the International Charter on Space and Major Disasters appears below.
Many natural disasters are caused by tsunamis. A United Nations (UN) report estimates that each year some 60,000 people and assets worth $4 billion are exposed to the threat of a tsunami. In addition, more than 700 million people are currently living in coastal areas at risk from extreme events such as tsunamis and storm surges. This number is likely to increase as coastal populations grow. The average tsunami wave is less than 10 feet in height, but some exceed 100 feet. It can produce extremely strong currents, quickly flooding the land and causing great destruction. These currents and floods can last for days. Since the beginning of the 20th century, 34 tsunamis have been recorded, collectively causing more than 500 deaths in the United States alone, and more than $1.7 billion in damage to US territories and coastal areas. The dangers posed by tsunamis emphasize the need for early warning systems – the longer the lead time, the more opportunities to evacuate and secure critical infrastructure.
Overview of Tsunami early warning systems
Currently, there are three technologies that can provide real-time early warnings of tsunamis. One of these is DART, a buoy-based technology. DART stations have many advantages, but they primarily serve as local and remote tsunami detection systems. In addition, they are portable, so the location of the arrays can be easily changed. Another advantage is the unified sensors, making it possible to share data with other countries in real time. Importantly, the buoys represent a distributed system, so one failure will not affect other DARTs.
Another technology is wired observatories, which are sensors located on underwater cable systems. The Joint Task Force on Monitoring Science and Reliable Telecommunications (SMART) Subsea Cables, a United Nations initiative, aims to equip new commercial undersea telecommunication cables with simple sensors that measure pressure, acceleration and temperature. The sensors can be added to fiber-optic cable signal boosters – watertight cylinders filled with equipment to amplify signals every 50 kilometers or so. With cabling that powers the sensors and transmits data, scientists can collect information about the seafloor on an unprecedented scale – and identify potential tsunamis much faster.
The image below depicts a surface buoy with a global positioning system (GPS) antenna docked about 20 kilometers off the coast to monitor sea level changes using real-time kinematic (RTK) GPS technology with a ground station. The buoys serve as a wave gauge to enable early detection of tsunamis. The real time GPS data is processed by PARI (Ports and Airports Research Institute), and then sent to the JMA responsible for tsunami monitoring and warning.
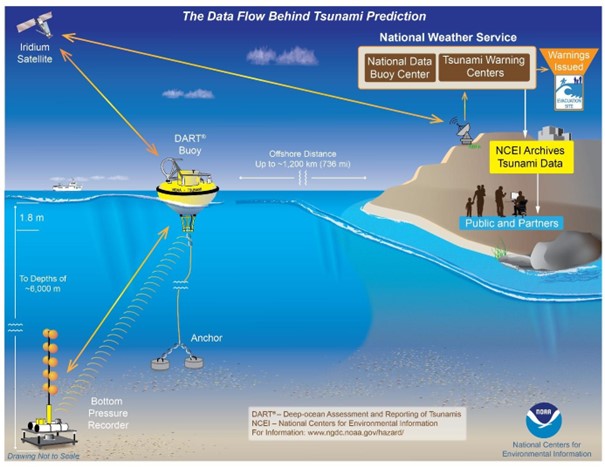
Tsunami early warning using Deep-ocean Assessment and Reporting of Tsunami, Source: NOAA
What are the limitations of these systems?
Unfortunately, despite their many advantages, these systems have a number of disadvantages, depending on the technology. In the case of DART, these include high emergency maintenance costs due to vessel-related expenses, and buoy locations must avoid strong ocean currents and seamounts. A surface buoy with a GPS antenna, on the other hand, requires a location close to the coast in order to communicate with GPS base stations. In addition, the sensors are non-standard, which makes the measurements incompatible with DART sensors and wired observatories.
However, regardless of the technology, early detection systems generally have two major limitations: late tsunami warnings and false alarms. A tsunami warning system is regarded as efficient if it provides enough time – referred to as “golden time” – for people to save themselves. The longer the golden time, the earlier the warning. Unfortunately, some countries still receive insufficient warning. “Tsunami warning systems are useless in most of the countries like Indonesia and Papua New Guinea, because the lead time is too short,” McCue told Reuters. “Far better to educate people to make for high ground immediately after they feel shaking that lasts more than about 30 seconds,” McCue said. The Pacific Ocean early warning system’s false alarm rate is estimated at 75 percent. “It’s definitely one of our biggest challenges,” said Charles McCreery, director of the Pacific Tsunami Warning System in Honolulu, which sends seismic data and tsunami bulletins to 26 nations around the Pacific. When a warning is issued, sirens sound on the beach, and urgent messages are displayed on television, and broadcast on the radio. Evacuation maps direct people to higher ground, but the tsunami often does not occur.
The Role of Remote Sensing Satellites in Tsunami Detection
How remote sensing satellites can detect early signs of tsunamis
How can orbiting satellites detect tsunamis at an early stage? Most tsunamis are caused by underwater earthquakes or volcanic eruptions, many of which produce atmospheric effects that satellites can easily detect.
An example is the recent eruption of the HTHH volcano in Vietnam, which ejected a volcanic plume that reached into the mesosphere and spread ash over a distance of several hundred kilometers. The satellite-detected atmospheric waves (Lamb) from this event spread around the world. The conjunctions of these atmospheric waves with ocean basins caused sea level fluctuations observed well before the tsunamis. For example, a tide gauge on Lord Howe Island, located about 3,000 kilometers southwest of the eruption site, recorded an initial sea level fluctuation of 0.1 meters about 2.5 hours after the eruption. Much larger waves of up to one meter followed, and the tsunami, moving at about 200 meters per second, arrived after another two hours. Atmospheric acoustic waves travel faster than tsunamis. Waves generated by extreme events, such as volcanic eruptions and tsunamis, penetrate the troposphere and stratosphere and reach as far as the ionosphere. Consequently, the upper layers of the atmosphere are ideal for detecting anomalies associated with these extreme events, since the amplitudes of atmospheric perturbations increase dramatically with altitude. Satellite technologies must be used to detect these atmospheric perturbations, providing high-resolution, wide-area information in the fastest possible time.
Satellites also provide early tsunami detection by monitoring the Earth’s crust for subtle changes in pressure, temperature and other factors, which may indicate an impending seismic event. In this way, a tsunami can be detected before it even occurs. In addition to detecting seismic activity, navigation satellites can also be used to provide data on ocean currents to predict the path and intensity of an approaching tsunami.
The advantages of using satellites for tsunami detection
Evacuation time is critical to minimize tsunami mortality rates. The sooner the threat is communicated, the greater the chance to minimize loss of life. A NASA Earth Applied Sciences project with NOAA has developed new technology for their tsunami early warning system to potentially predict tsunamis within just five minutes of an earthquake, which could significantly affect the number of fatalities. “The problem we’re trying to solve is that it’s difficult to produce a tsunami forecast within the first 20 minutes that says when and where a tsunami might occur, or how big the waves might be,” said Diego Melgar from University of Oregon, who leads the project’s research team in the NASA Disasters Program area. “That’s where GNSS [Global Navigation Satellite System] comes in – it can measure tectonic displacement within the first minute, determine the magnitude of the earthquake, and trigger an alert to the region within those critical first five minutes.” Melgar’s team also uses real-time data from Global Differential Global Positioning System. This helps to provide more accurate and immediate estimates of tsunami size and direction. “Incorporating this data from NASA means we can enable emergency response managers to provide actionable tsunami impact information to their communities sooner, giving local residents as much time as possible to take life and property saving actions,” Angove said.
Examples
An earthquake measuring 9.0 on the Richter scale, the strongest ever recorded in Japan and the fourth strongest since 1900, occurred in the Pacific Ocean near the Tohoku region of Japan. The earthquake and subsequent tsunami caused massive damage along the Tohoku-Kanto Pacific coast. The Japan Aerospace Exploration Agency (JAXA), together with Sentinel Asia and the International Charter on Space and Major Disasters, planned satellite observations of areas immediately after the disaster. The images acquired were used to assess the overall extent of damage, analyze tsunami-inundated areas, landslides, damage to structures, road accessibility and shelter locations. In addition, thanks to the satellite images, SAR rescue workers had knowledge of the availability of key facilities and rescue routes, which contributed to a more efficient evacuation. The use of satellites has proven the unrivaled speed of relief efforts during mass disasters.
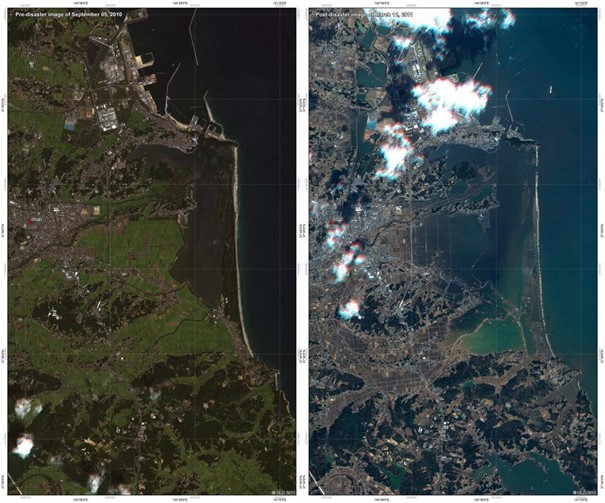
Japan’s changed landscape from space, Source:
https://www.esa.int/About_Us/ESRIN/Mapping_Japan_s_changed_landscape_from_space
Satellites were also helpful in the December 2004 Indian Ocean tsunami. About two hours after the initial earthquake, Jason, the joint U.S.-French satellite took measurements of sea surface elevation and waves to study ocean circulation and climate. The maximum sea surface elevation of 50 cm was measured about 1,200 km south of Sri Lanka, where the leading wave length was about 800 km, followed by another with a crest of 40 cm. Near the northern end of the Gulf, two waves of 40 cm and 20 damaged the coast of Myanmar. Topex/Poseidon, simultaneously orbiting about 150 kilometers west of Jason, made similar observations of tsunami waves, confirming that the measurements were consistent. “The observations made by Jason and Topex/Poseidon are unique and of tremendous value for testing and improving tsunami computer models and developing future tsunami early warning systems,” said JPL’s Dr. Lee-Lueng Fu, Jason and Topex/Poseidon project scientist.
Conclusion
Remote sensing satellites provide great support in early tsunami warning. Current technologies, while not yet perfect, make it possible to predict a natural disaster before high waves are even captured at sea. This provides more time for evacuation, minimizing loss of life and damage to infrastructure.
Fast availability of data enables more efficient management of emergencies, where every minute counts. However, new solutions usually present challenges. Satellite imagery is expensive, and the cost of acquiring and processing the data can be prohibitive for some researchers. The second issue is the need for international cooperation. For example, the cooperation between different organizations during the 2011 floods in Japan enabled more effective action, which is crucial when dealing with natural disasters. Remote sensing satellites have enormous potential for early detection of tsunamis, and to reduce the damage and loss of life these disasters cause.
Did you like the article? Read more and subscribe to our monthly newsletter!