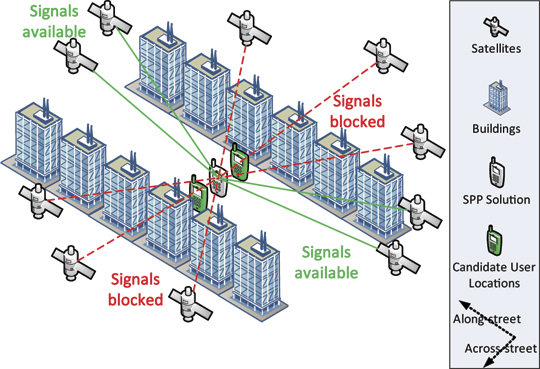
GNSS Shadow Matching: Improving GNSS positioning in Urban Canyons
In the era of smartphones and smart devices, navigation in urban areas still remains a gray area where position accuracies (of around 5m) can be frustrating and misleading. Certainly all of us have experienced being misled to take a wrong turn because of this urban canyon problem. It’s amazing how 5 meters can make such a difference!
Why is the accuracy so bad in urban areas?
The reason for poor GNSS positioning accuracy is due to the lack of direct line-of-sight (LOS) signals from GNSS satellite constellations. Without direct signals, our smartphones and navigation devices are forced to use signals that have multipath in them. Despite many algorithms that totally neglect signals with multipath or use corrections, the accuracy is still not comparable to conditions where LOS signals are available. Although, Using GLONASS in addition to GPS does considerably enhance the availability of LOS signals it is still not going to solve the problem that arises due to signal geometry.
We are surrounded by buildings that are parallel to the street, which means we are more likely to receive LOS signals from satellites that are along the satellite than across the street. This leads to position accuracy to be better along the street than across it. The end result is that, we will know accurately whether we are standing near door number 10 or 15 but we will have issues knowing whether it is the first street or the second.
How to solve this urban canyon problem in GNSS?
Well, we first need to accept the reality that so far we have not been able to come up with a better solution than GNSS to locate ourselves accurately on a global scale which means any solution that we think of, has to be able to take GNSS positioning as an input and better it; A solution that acts as a feedback loop mechanism rather than acting as a stand-alone solution.
Obviously we could then use INS (inertial navigation systems), iBeacons, WiFi signals, Mobile triangulation technique and maybe hundred other options but the technique that caught my attention – “GNSS Shadow Matching”! The GNSS Shadow Matching technique is currently being developed at the University College London by Dr. Groves and his team.
Improving GNSS positioning in urban canyons requires lateral thinking. If it’s not possible to calculate a sufficiently accurate position solution using the visible satellites, why not use the non visible satellites as well? This is exactly what shadow matching does. If you know where the buildings are and how big they are, you can deduce positional information from the knowledge that certain signals are blocked. – GPSWorld
The GNSS shadow matching technique makes use of 3D models of a city to accurately pinpoint your location. The principle behind shadow matching of GNSS signals is quite simple and straightforward, you know the building in front of which you are standing, thanks to 3D Building models and Image Processing. It is also possible to determine which satellites will have direct LOS to this building at any given point in time. We combine these two to determine our position with much better accuracy. Match the “shadow” signals with the actual signals that we are receiving and immediately our position accuracy is set to improve.
Quite Interesting right? Well, I cannot imagine using this technique tomorrow morning to navigate because of a couple of reasons, 3D models are not as widely available as much as we would like them to be. The drain on my poor smartphone battery if I have keep my camera turned on all the time. In conclusion, we still have to rely on using WiFi and Mobile Triangulation techniques to solve our urban navigation headache. However the GNSS Shadow Matching technique is still in its nascent stages and it would be foolish to write it off already. Who knows what the future holds in store 😉
If you want to read more about this interesting technique, here’s the link to the complete research article that also featured on the INSIDEGNSS magazine.
Did you like this post on GNSS positioning? Read more and subscribe to our monthly newsletter!
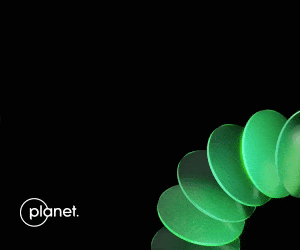