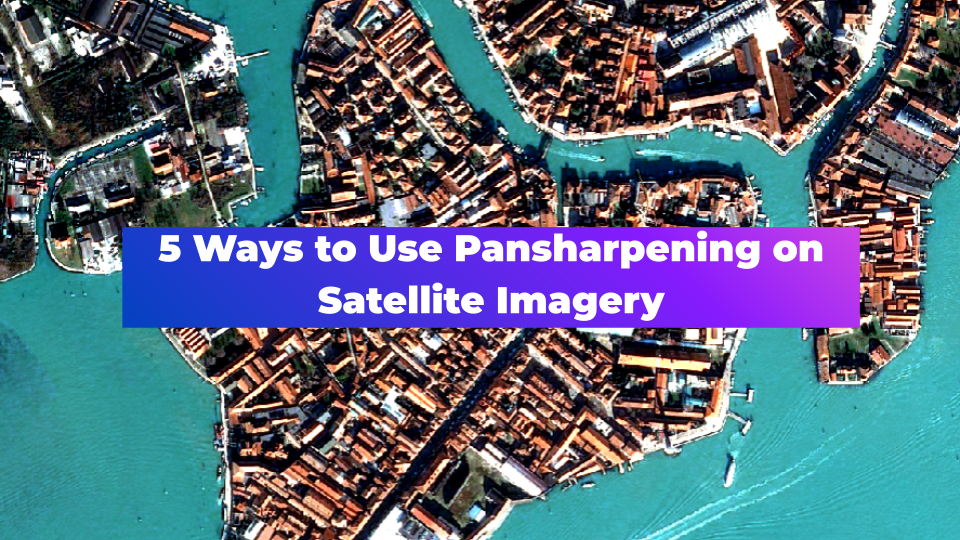
5 Ways to Use Pansharpening on Satellite Imagery
Editor’s note: This article was written as part of EO Hub – a journalistic collaboration between UP42 and Geoawesome. Created for policymakers, decision-makers, geospatial experts and enthusiasts alike, EO Hub is a key resource for anyone trying to understand how Earth observation is transforming our world. Read more about EO Hub here.
The topic of pansharpening has been covered far and wide. Lots has been said about it, so in this article, we’re going to let the images speak for themselves. But first, here’s a whistlestop tour of pansharpening: what it is, its origins, how it works, and five ways to use it.
What is Pansharpening?
Both well-loved and well-used, pansharpening (short for panchromatic sharpening) is an image fusion technique used to enhance satellite imagery.
The nature of remote sensing satellites means that they only capture high spatial resolution or high spectral resolution imagery.
Imagine you only have two photos of the same scene:
- A very sharp black-and-white photo (panchromatic).
- A less detailed but colorful photo (multispectral).
That’s where pansharpening comes in.
It solves two main problems at once, which are:
- The panchromatic band has low spectral resolution and high spatial resolution.
- Multispectral bands have high spectral resolution and lower spatial resolution.
Pansharpening combines these two images to create a single picture that has the best of both worlds.
The result? A high spatial, high spectral resolution image.
This allows us to see the planet’s features more clearly, making imagery easier for both humans and computers to analyze and understand.
The History of Pansharpening
The roots of pansharpening emerged in the 1970s-1980s as satellites began capturing both panchromatic and multispectral imagery. Early research focused on combining high-resolution panchromatic data with lower-resolution color information.
Key techniques like IHS transform and Brovey transform were developed in the 1990s, leading to the widespread adoption of pansharpening in the 2000s with high-resolution commercial satellites.
How does Pansharpening Work?
Pansharpening using the Intensity-Hue-Saturation (IHS) method is a popular technique. Here’s how it works:
Step 1: Convert Multispectral Data to IHS Color Space
Multispectral imagery typically includes three bands (e.g. Red, Green, Blue), which have lower resolution compared to the PAN band. The first step to enhancing resolution involves converting the RGB imagery into a new image with PAN-based resolution. Then, this resampled RGB image is converted into an IHS model.
What’s IHS?
- Intensity (I) represents brightness.
- Hue (H) corresponds to the dominant wavelength (perceived color).
- Saturation (S) indicates how vividly these colors appear.
Separating these elements allows us to process spatial information (brightness) and spectral data (color) independently.
Step 2: Merge with a Panchromatic Image
The low-resolution Intensity component is replaced with the high-resolution panchromatic image. By substituting the Intensity component of the multispectral image with a high-resolution panchromatic image, we achieve enhanced spatial detail. The panchromatic image, which has finer spatial resolution than the original intensity layer, effectively boosts the clarity of the final image. The color data—hue and saturation—remains untouched, preserving the spectral richness while upgrading the sharpness.
Step 3: Transform IHS to RGB
Finally, after merging the panchromatic data, the new high-resolution IHS image (with new Intensity) is converted back to RGB. This step restores the color richness from the multispectral data, now combined with the improved spatial detail from the panchromatic layer.
The result? A visually stunning high-resolution color image that offers both enhanced detail and vibrant, accurate colors.
Did you know you can now pansharpen your scenes directly on the UP42 platform? Provided by Pixel Factory, the algorithm allows you to process high-quality Earth observation products using the Brovey method.
You can find more information about the requirements, input, and output in UP42’s technical information. Try pansharpening out for yourself on UP42, for a quick and easy way to extract insights at scale.
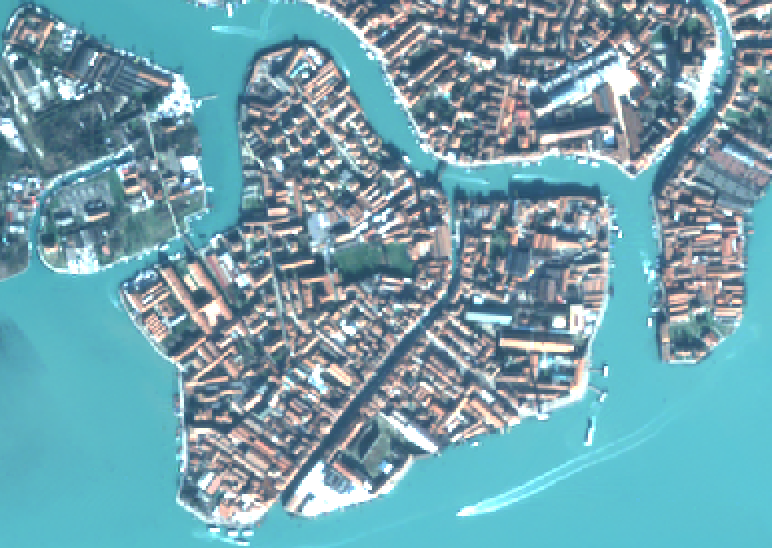
Pléiades, Airbus, original image
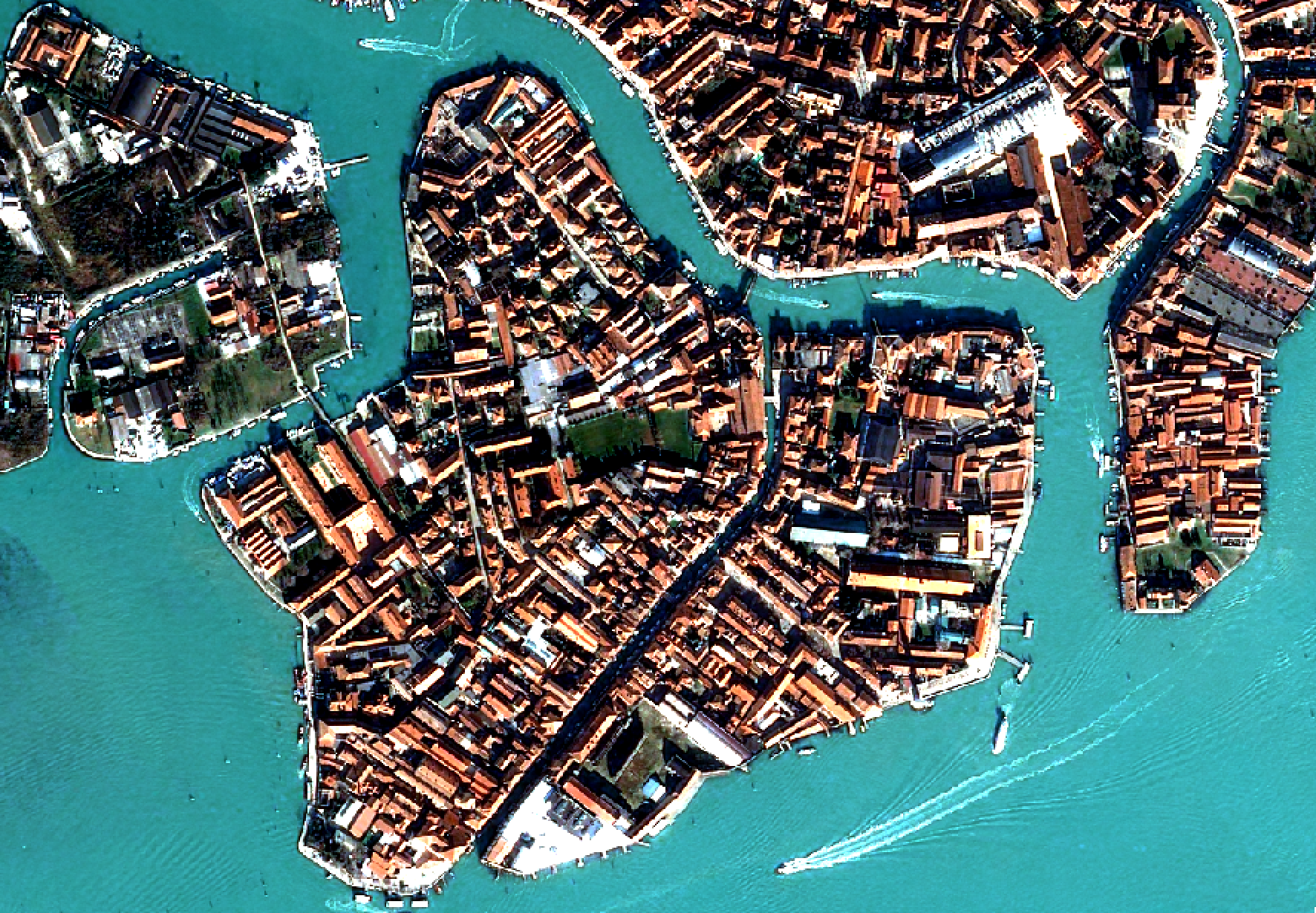
Pléiades, Airbus, pansharpened
How Can We Use Pansharpening?
Now, onto the images. We’ve zoomed into areas around the world – Berlin, Anak Krakatau, Prignitz, State of Pará, and Boxmeer – to give you an idea of how useful pansharpening is across use cases and the globe.
Our workflow looked like this:
- Source data: Landsat
- Bands: 2 (Blue),3 (Green),4 (Red),8 (Panchromatic)
- Tool: GDAL Pansharpening
- Aim: Visualize a raster with 30m resolution into a raster with 15m resolution
- Locations:
Berlin, Germany: Urban Areas
First, we looked at Berlin, Germany. It’s an ideal example of an urban area and the impact that pansharpening can have. In the after image, we’re better able to distinguish between closely spaced objects like individual buildings, and roads.
Pansharpening significantly improves the detection of the fine details of urban structures and buildings, which may not be visible in lower-resolution imagery.
Object detection algorithms perform better when used on pansharpened imagery, as well as other automated analysis techniques. This makes pansharpened imagery particularly valuable for urban planning purposes, such as mapping out population trends, urban land use and land cover changes, and supporting decision-making.
Anak Krakatau, Indonesia: Landslides and Tsunamis
The December 2018 landslide and tsunami in Atak Krakatau, Indonesia drastically reshaped the volcano and surrounding coastal areas. The volcano collapsed into the sea, triggering a devastating tsunami, reducing the island’s height by over 50% and resulting in hundreds of lives lost and casualties.
By applying pansharpening to satellite imagery of Anak Krakatau, we gain a more detailed picture of the changes. Pansharpened data for natural disasters could lead to better risk assessment, disaster response planning, and understanding of similar events in the future. Also, it’s highly useful for volcano activity monitoring and aftermath events in frequent temporal resolution.
Prignitz, Germany: Green Energy and Farming
Pansharpening satellite imagery of Prignitz, Germany – an area known for its farming fields and wind turbines – can provide significant benefits for green energy applications and related use cases.
When it comes to planning new wind farms, pansharpening is a game-changer. It’s great for seeing the lay of the land – where the hills are, how close the turbines would be to residential areas, how many wind turbines are there, and what condition they’re in. Images with PAN resolution are perfect for detecting engineering objects and monitoring the construction process, which often requires more pixels for accurate analysis.
In general, pansharpened imagery enhances the ability to detect changes over time. This means better long-term monitoring for both wind farm expansion as well as agricultural development. As you can see in the images of Prignitz, it’s clear how pansharpened satellite imagery helps to keep an eye on the environment.
State of Pará, Brazil: Deforestation
Next, we looked at a critical area of concern for deforestation in the Brazilian Amazon. In 2023, Pará accounted for 36.4% of the total deforestation in the Brazilian Amazon, making it the state with the highest deforestation rate.
As you can see in the images below, there is much clearer visualization in the pansharpened version. This helps with discerning forest boundaries, forest cover changes, and natural forest regrowth. Thanks to this, you can also observe illegal logging more closely – noticing small gaps in the forest cover.
The deforestation rate in Pará has remained high despite overall reductions in Amazon deforestation. For instance, even when Brazil achieved an 84% reduction in Amazon deforestation in 2012 compared to 2004 levels, Pará continued to be a major contributor to forest loss. The enhanced visual interpretation, improved automated analysis through machine learning algorithms, and temporal monitoring that pansharpening offers make it a key step toward tackling deforestation.
Boxmeer, Netherlands: Flooding
Boxmeer is a town in upper southeast Netherlands, which experienced flooding during extreme weather across Europe in 2021. We’ve pansharpened imagery of the area, which highlights the difference pansharpening makes.
For flooding, pansharpened satellite imagery can be extremely valuable for monitoring and managing risks, especially in the Netherlands. The country’s historic battle with flooding, combined with climate change and heavy rainfall, means that risk mitigation is key. Over 50% of the Netherlands is below sea level, making the country particularly vulnerable to flooding. Pansharpened satellite imagery can significantly enhance countries such as the Netherlands’ ability to monitor, respond to, and plan for flooding.
The aftermath of this catastrophic event was focused on a river bank erosion assessment to protect critical places against further potential floods.
Now that we’ve seen what pansharpening can do, it’s incredible to see how far we’ve come with satellite imagery. What began as a clever way to make photos clearer has evolved into a whole field of space tech.
Today, we’ve got AI and advanced machine and deep learning approaches working their magic to find new ways to improve image quality and reduce spectral distortions. The future of Earth observation is looking bright – and super high-res!
Did you like the article? Read more and subscribe to our monthly newsletter!
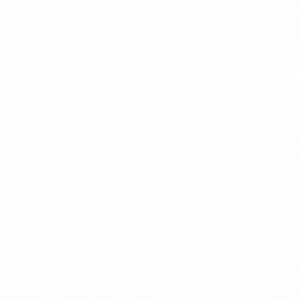