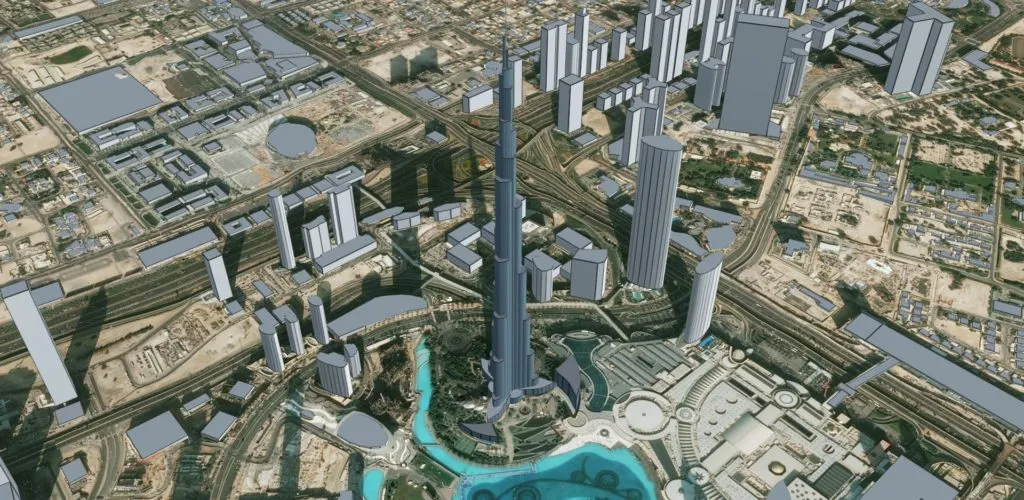
Cesium now supports OSM layer of over 350 million 3D buildings
Cesium – a startup dedicated to making 3D geospatial data accessible, shareable, and ultimately usable – has just added a new global base layer that promises to make urban mapping applications built using the Cesium visualization platform much more engaging.
Cesium OSM Buildings is a 3D buildings layer covering the entire world built with OpenStreetMap building data. That means more than 350 million buildings with incredibly rich metadata – think building names, building type, address, opening hours, building height, and even the shape of the roof in some cases!
Available as a 3D Tileset on Cesium ion, OSM Buildings could prove to be a great base to layer high-resolution data on and provide immersive urban experiences. Since the data is crowdsourced and updated monthly, cities, governments, or even builders should be able to create numerous shareable 3D solutions using Cesium OSM Buildings.
Also see: Cesium builds 3D data visualization app for construction giant Komatsu
For example, the base layer could be used for urban planning, tax assessment, or traffic management. BIM models of construction projects could be visualized with a real-world context. Government agencies could use it as an innovative tool for training and simulation. And with per-building metadata, you could even highlight all commercial and residential buildings to see patterns in different neighborhoods across a city.
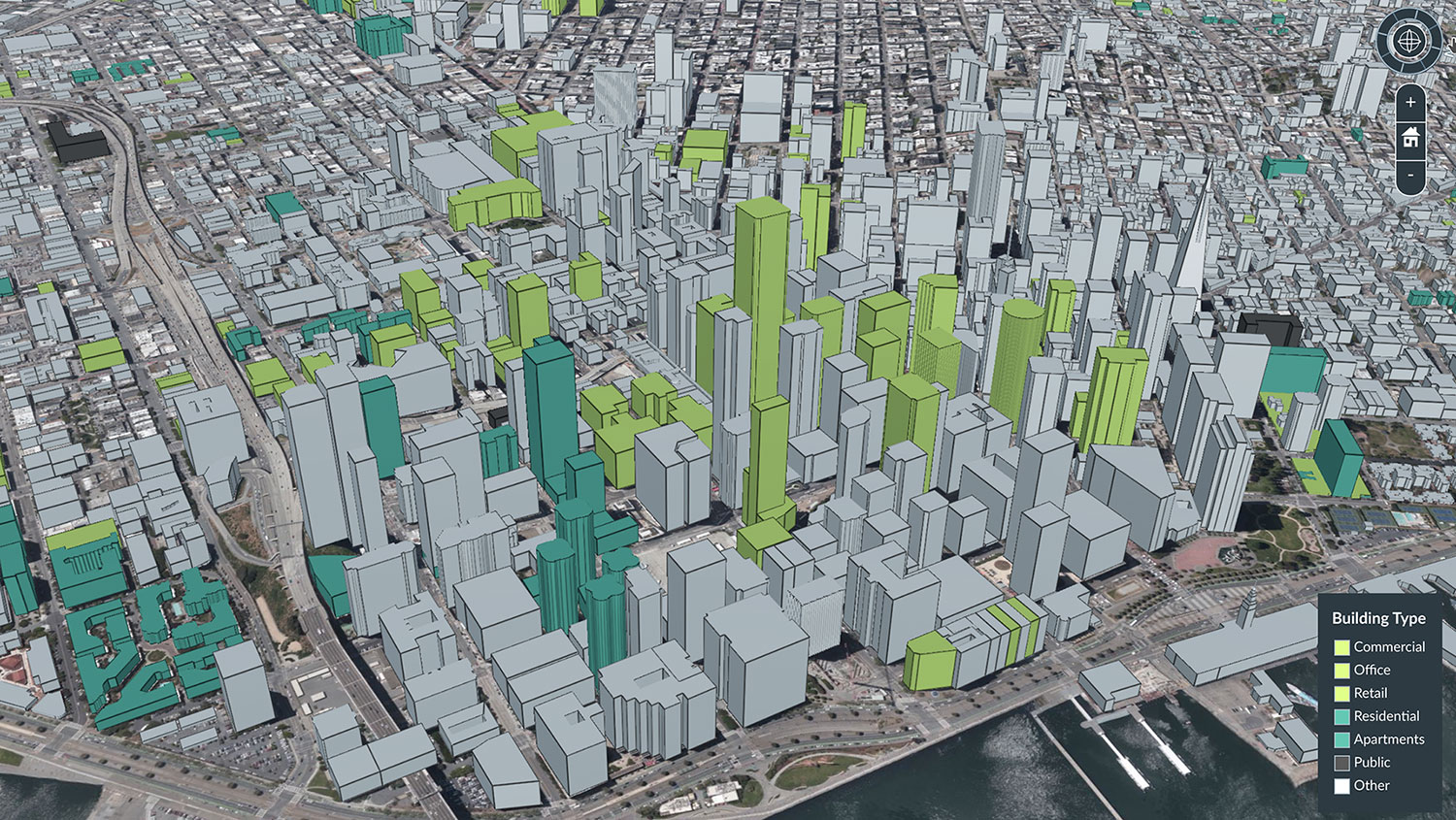
Highlighting all offices and commercial buildings in green using Cesium Stories
The full dataset is hundreds of gigabytes in size. But when you use it with Cesium 3D Tiles, the data can be streamed efficiently and visualized over the web. 3D Tiles is an open standard, so Cesium OSM Buildings can be used in any compatible viewer, in addition to the open-source CesiumJS.
Try out the data here.
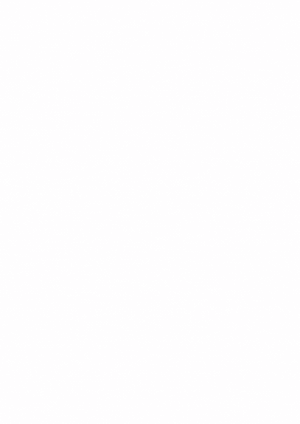
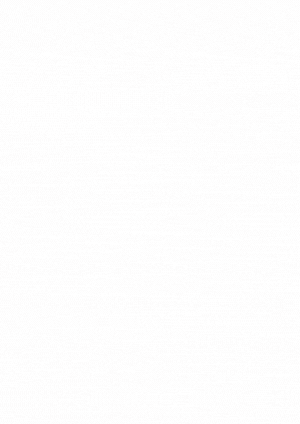