Building Together an Open Collection Of Historical Maps: Discover the Wikimaps project
The biggest challenge of our times regarding geographic information is to process and organize a massive amount of data from various sources, with various dates and especially, formats. Big data is about processing a huge and diversified volume of information, with high velocity and high relevance – which is what Google pretty much succeeded to do so far with the internet.
How about geographical information? How about the huge amount of maps, data points, places, layers of thematic information all the villages, cities, private corporations, land authorities, NGOs and other organizations have put together for centuries? How about this extremely rare and unique knowledge everybody has in their basement without even knowing it is here?
[The Wikimap Project Facebook Group]
Galleries, libraries, archives and museums (the GLAMs) are the keepers of this unsuspected information, for the common good. How about we – you and me, the average citizen – succeed to dig out, put together, standardize and classify this dusty pile of historical maps and place related data and turn it into an open, free and smart online catalogue?
That is the goal and ambition behind wikimaps, the wikipedia project to collect historical maps. It might look simple, but there is a serious technical challenge behind it. Every company in the world is currently struggling to find solutions to keep velocity and relevance going while collecting trillions of data per minute. Every company is trying to find highest the added value in the mess (and masses) of data to improve their product and open new ways of consuming and informing. The spirit is more knowledge-oriented than business-minded regarding the wikimap project, but that is one more great initiative that will definitely contribute to collect and order our geographical legacy.
One example of the hidden treasures we can find in archive and their impact on our understanding of the world: In September of 1861, the U.S. Coast Survey published a large map, just under three feet square, titled a “Map showing the distribution of the slave population of the southern states of the United States.” Based on the population statistics gathered in the 1860 census, and certified by the superintendent of the Census Office, the map depicted the percentage of the population enslaved in each county. Also, one interesting slideshow to know more about the project:Wikimaps is a project where wikimedians work together with GLAMS (Galleries, Libraries, Archives and Museums) to collect old maps in Wikimedia Commons. The project will offer tools for georeferencing the maps via crowdsourcing, and make them available for historical mapping with the OpenHistoricalMap project. The information gathered from the maps will contribute to a historical gazetteer, a place name index across times.
Currently an enormous amount of maps are locked in archives. They have not been digitized, they are behind cumbersome user interfaces or copyrights limit their use. In most cases the map is just an image and does not align with real world coordinates. The Wikimaps project hope to change that!
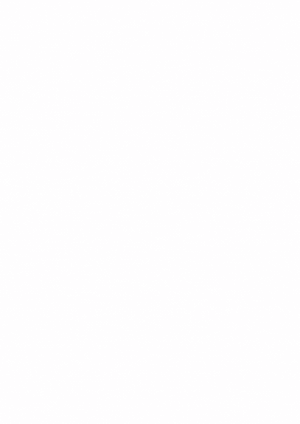