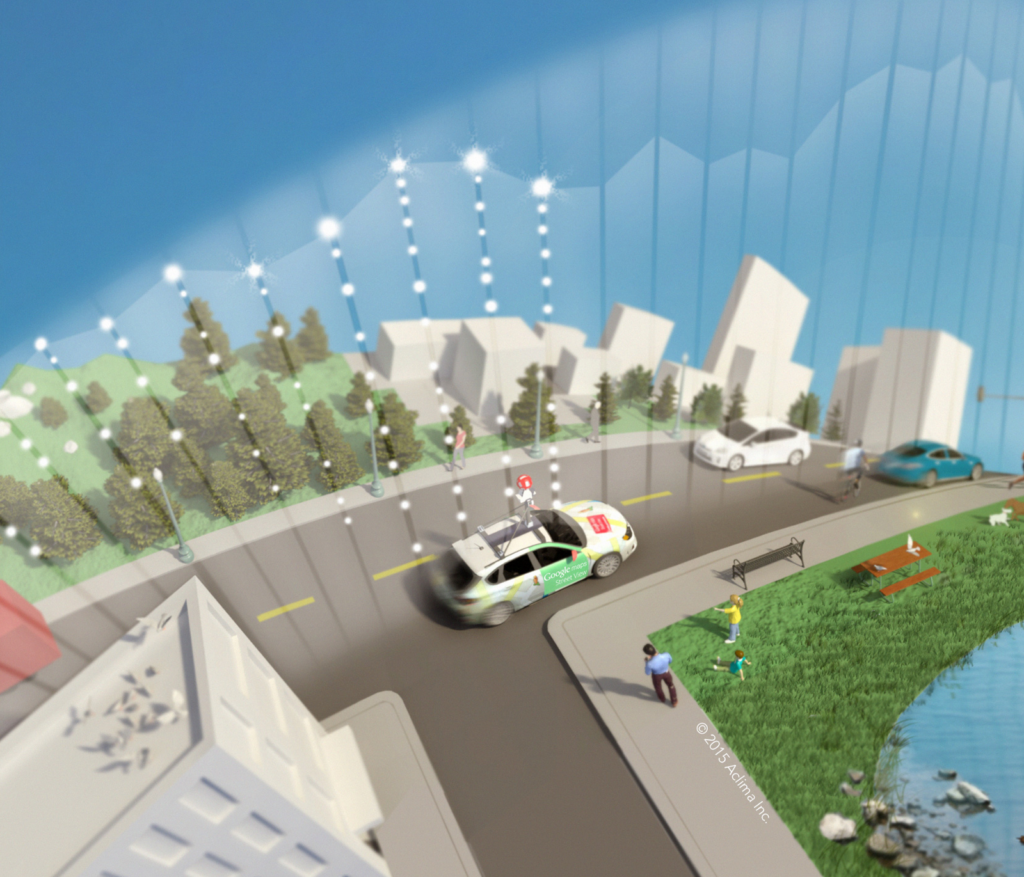
Aclima to scale intelligent air pollution mapping platform with $24M funding
Remember Aclima, the tech startup helping Google map air pollution in places like San Francisco and London? Well, it just raised a cool $24 million in Series A funding to expand its air quality monitoring program.
Aclima’s environmental monitoring sensors – fitted on the roof of Google’s Street View cars and planted on stationary objects like buildings and lampposts – have been gathering millions of hyperlocal air quality data points in various cities. This includes information about emissions like carbon dioxide, particulate matter, carbon monoxide, methane, black carbon, ozone, total volatile organic compounds, etc.
Davida Herzl, founder and CEO of the California-based startup, explains that this funding will be used to scale up and advance both mobile and stationary mapping of hyperlocal air pollution and emissions in various cities. She says, “This funding will accelerate Aclima’s efforts to both fill a critical gap in air pollution and emissions data, and transform this new class of information into environmental intelligence that drives better decision-making for communities, cities and the enterprise.”
What has worked in Aclima’s favor is that the startup has found a way to reduce the cost of traditional air pollution measurement methods significantly. Its platform uses machine learning to deliver high-resolution emission maps with up to 100,000 times greater spatial resolution than conventional sources.
So, it’s not really surprising that Aclima has been rubbing shoulders with organizations like Google and the US Environmental Protection Agency (EPA). Climate risk management is a pressing issue for all governments, and having access to hyperlocal emissions data and pollution insights is a critical component for making informed decisions.
Last year, Aclima released the results of its year-long mobile mapping campaign in Oakland, California, which found that air pollution could vary from block to block along the same city street. This study, published in Environmental Science & Technology, highlighted the importance of identifying local pollution hotspots in order to understand their impact on human health and environment.