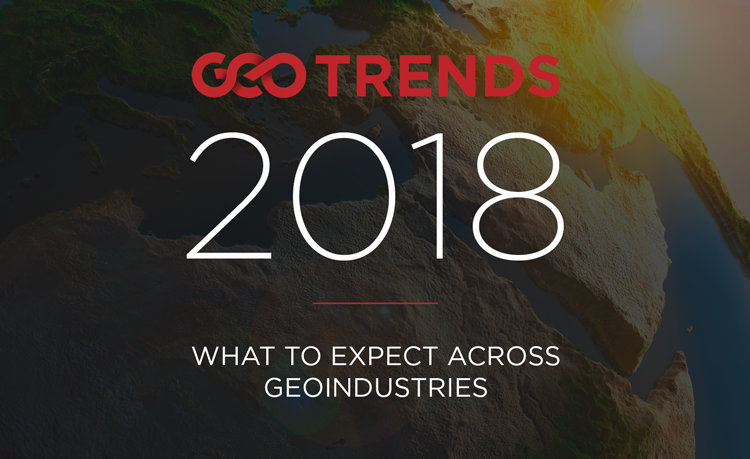
5 ways geolocation will change marketing in 2018
Advertisers have had the option to target search and social media ads based on geographic locations for some years now. But, when Pokémon Go came out of nowhere in 2016 and became an instant global phenomenon, it represented a watershed moment for location-based marketing opportunities. Brands discovered that geolocation technology could not only help them find out where their customers are, but also the context of their visit to that place.
Not surprisingly, last year, we saw Facebook announcing it will show you ads based on the stores you have visited in person, and Snapchat’s parent company Snap acquired location analytics firm Placed to show advertisers how online ads lead to offline store visits.
Javier de la Torre, the CEO of location intelligence platform Carto, tells Geoawesomeness he’s positive marketing campaigns based on consumer proximity and daily habits will become standard practice in 2018. “Data about where consumers eat, shop and socialize is increasing, and marketers will continue to lean on these insights to tailor data-driven advertisements based on real-time location.”
So, what are some ways in which we can expect geolocation to dominate the world of advertising and marketing? Let’s find out…
1) Augmented marketing and events
Augmented reality (AR) relies on a person’s actual location in the real world to overlay additional computer-generated information on top of it, to create a virtual world of sorts. Several brands like L’Oreal, Tesco, and Sephora are using AR for their advertising and marketing initiatives. At the recent North American International Auto Show in Detroit, Ford used AR to show attendees the technology under the hood of its vehicles in an X-ray vision of sorts.
2) Turbocharged predictive analysis
Give predictive algorithms a dose of location and you can take behavioral insights to another level. If advertisers are aware of the route a user normally takes or if they can see a certain area which the customer frequents (like an ice rink or a nature park), they can send targeted adverts at a time when they would be most impactful.
3) Ads kindled by indoor mapping
With map providers, major retail locations, airports, and other large avenues pushing for comprehensive indoor maps, expect to see more deals or discounts from advertisers pop up while you are passing by their stores. Apart from allowing advertisers to push out timely communication, geolocation allows gives them invaluable access to the customer behavior.
4) Weather-based geo advertising
Geolocation-based weather triggers give marketers an unprecedented opportunity to send out personalized messages to their target base. For example, on a particularly hot day, a beverage company can invite customers in for a refreshing iced tea. Or if the forecast predicts heavy showers next week, an outerwear business can offer deals on raincoats. Expect to see more such targeted advertising this year.
5) Digital influencers for local retailers
Finding digital influencers from around the globe is not a difficult task. It only takes a quick Google search. But, a national-level influencer program will not provide the desired return on investment to a local or regional retailer who wants to target the nearby community. Geolocation is now helping retailers to find relevant influencers based on geographic context as precise as their own street level.
Have you seen a brand using location-based marketing in a unique manner or is your business leveraging geolocation to bring in customers? Share your story in the comments section below!