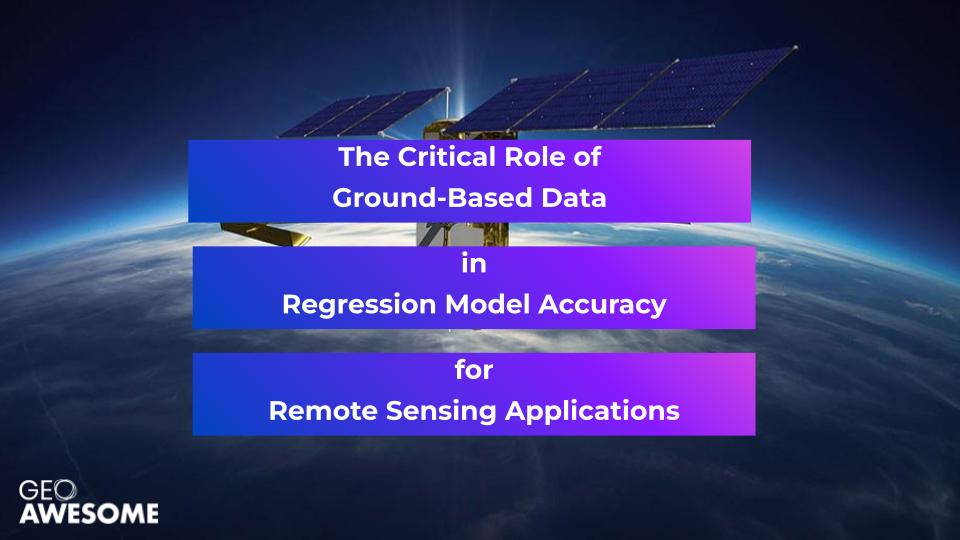
The Critical Role of Ground-Based Data in Regression Model Accuracy for Remote Sensing Applications
Editor’s note: This article was written as part of EO Hub – a journalistic collaboration between UP42 and Geoawesome. Created for policymakers, decision-makers, geospatial experts and enthusiasts alike, EO Hub is a key resource for anyone trying to understand how Earth observation is transforming our world. Read more about EO Hub here.
Remote sensing has revolutionized environmental studies, allowing scientists to gather comprehensive data about the Earth’s surface and atmosphere from afar. From monitoring deforestation and mapping urban sprawl to assessing agricultural health and predicting natural disasters, the applications of remote sensing are vast and varied.
However, despite these technological advances, the accuracy of the regression models used in remote sensing heavily relies on ground-based data. This article delves into the indispensable role of ground-based data in calibrating and validating these models, thereby ensuring the reliability of remote sensing analytics.
Pixel-Level Detail: Categorizing Imagery with Labels and Values
Since the launch of Landsat-1 in 1972, satellite imagery has become increasingly accessible, marking over five decades of open-access satellite imagery covering the globe. With a spatial resolution of 30 meters, the Landsat constellation provides moderate-resolution imagery, enabling applications ranging from land cover classification to environmental monitoring. This resolution allows us to observe landscape features at a human scale while still covering large areas.
Today, multiple sources of open-access imagery, such as MODIS and the Sentinel constellation, as well as commercial options providing sub-meter spatial resolution, are available. These datasets are now easily accessible through platforms like UP42. Increased accessibility has propelled a wide range of applications, particularly in image classification. You’re now able to efficiently assign a class label to each pixel, such as:
- Water bodies
- Vegetation types
- Snow and ice cover
- Urban structures and buildings
- Agricultural fields
From Classification to Regression
Recently, there has been an increasing shift towards using satellite imagery for regression modeling. Instead of assigning a label, regression modeling assigns a quantitative value to each pixel. This is a shift from traditional semantic segmentation, which assigns class labels like ‘building or ‘nonbuilding’. Instead, regression models predict a continuous quantitative value for each pixel, such as crop yield or vegetation indices. This approach allows for:
- More granular information extraction
- Capturing subtle variations within a single land cover type
- Direct quantification of physical or biophysical parameters
Some key applications of regression modeling include estimating forest biomass, examining soil moisture, and looking at water quality parameters. For example, pixel-level regression is ideal in precision agriculture for predicting crop yield. It’s useful in urban planning for estimating building footprint height and can be used in environmental monitoring when quantifying vegetation indices.
A quick review of remote sensing studies indicates that a significant portion of studies focus on classification and change detection, whereas the applications using regression modeling are still being explored.
This trend toward pixel-level regression is driven by several factors:
- Demand for Granularity: There’s an increasing need for more detailed, quantitative information beyond simple categorical labels
- Richer Information: Regression models can provide more nuanced insights into continuous variables like vegetation health, soil moisture, or urban density.
- Improved Decision Support: Quantitative data often translates more directly into actionable insights. the need for more granular and quantitative information, beyond categorical labels.
While promising, the shift towards regression modeling in remote sensing comes with its own challenges. Regression models typically require more finely annotated training data, with continuous ground truth values for each pixel.
This is where ground-based data comes in.
The Critical Role of Ground-Based Data
Ground-based data, or “ground truth” data, is essential for validating and calibrating remote sensing observations. Collected directly from the Earth’s surface, it excludes atmospheric effects and other errors, ensuring accuracy in regression models. Key reasons for its importance include:
- Training Data: Proper calibration with field data minimizes errors. For instance, satellite sensors might misinterpret different surfaces. Ground data helps correct these inaccuracies.
- Model Validation: Validation with ground data ensures predictive accuracy. For example, crop yield models must be validated against actual field data to refine and improve reliability.
- Spatial Extrapolation: Ground data allows model predictions to be applied over larger areas. A model calibrated with soil moisture data from one region can estimate moisture levels in similar regions without direct measurements.
- Data Integration: Integrating ground data with high-resolution imagery from UAVs enhances the validation database, improving model accuracy. For example, combining groundwater quality measurements with UAV data enhances large-area predictions.
- Physical Interpretability: Ground data provides context, making models more transparent and their predictions more understandable.
- Error Correction and Bias Adjustment: Ground data helps identify and correct biases in satellite data, such as adjusting satellite-derived temperature estimates with ground-based readings for better accuracy.
Sources of Ground-Based Data for Remote Sensing Validation
Ground-based data plays a crucial role in calibrating and validating remote sensing models. Two primary sources of this data are field measurements and reflectance measurements.
- Field Measurements: These involve on-site data collection using instruments to measure various environmental parameters, such as soil moisture, vegetation type, and atmospheric conditions. For instance, scientists may use on-site turbidimeters to check the turbidity of the water. Additionally, samples collected from the field can be stored carefully and brought to the laboratory for analysis, such as chlorophyll concentration or the amount of nutrients in the water or soil sample.
- Reflectance Measurements: It is important to collect the reflectance spectra as satellite data is affected by atmospheric composition, these measurements help account for those atmospheric effects—providing a direct link between ground conditions and remotely sensed data.
Reflectance measurements are typically taken using a handheld spectrometer that provides hyperspectral reflectance values (typically from 390nm to 900nm with 1nm bandwidth).
Using Regression Models in Remote Sensing
Now that we have established the importance of ground-based or “in-situ” data for remote sensing applications, it is important to understand how to use regression models and their need. Put simply, regression models are statistical techniques used to predict continuous variables based on the spectral data obtained from satellite imagery.
Inputs for Regression Models
Regression models can utilize one or a combination of inputs:
- Original spectral bands such as Blue, Red, Green, etc.
- Band ratios and indices such as Blue/Green, or NDVI (Normalized Difference Vegetation Index)
- Spectrally transformed values such as PCA (Principal Component Analysis) and (Hue, Saturation, and Value)
- In-situ parameters, for instance, turbidity or suspended particulate matter, can be used in the estimation of water clarity or chlorophyll-a
- Environmental and meteorological parameters such as rainfall, air and water temperature, wind speed and direction
Thankfully, we do not have to manually calculate all these indices as platforms like UP42 offer processing tools for calculating various indices and processing data, streamlining the workflow for regression modeling.
How Do These Models Work?
Regression models establish relationships between dependent variables (e.g., soil moisture, and biomass) and independent variables (e.g., spectral bands from satellite imagery). The goal is to predict the value of the dependent variable for each pixel in an image based on the spectral information. Common types of regression models used include:
- Linear Regression: Establishes a linear relationship between the dependent and independent variables.
- Multiple Linear Regression: Involves more than one independent variable to predict the dependent variable.
- Non-linear Regression: Captures more complex relationships that are not linear, such as polynomial, logarithmic, or exponential.
- Machine Learning-Based Regression: Methods such as Random Forest, Support Vector Regression, and Neural Networks can model complex, non-linear relationships more effectively than traditional regression techniques.
Why Are These Models Needed?
Regression models are crucial for transforming spectral data into meaningful environmental parameters. Unlike classification models, which assign a predictive value to each of the satellite-based pixels and not a class label, regression models can be used to accurately map and monitor an environmental variable on a large spatial and temporal scale.
Challenges in Using Ground-Based Data
- Remote areas and difficult terrain hinder data collection, making it logistically and physically demanding.
- High costs for specialized equipment, travel, and personnel make extensive data collection expensive.
- Temporal discrepancies between ground data collection and satellite overpasses, along with natural environmental changes, lead to potential alignment issues.
Alternative Approaches
Given these challenges, researchers are exploring alternative methods to supplement ground-based data. High-resolution imagery, drone-based data, citizen science initiatives, sensor networks, and data fusion techniques, all mitigate some of the challenges associated with ground-based data collection. Platforms like UP42 offer access to a diverse array of geospatial data sources, enabling users to combine data types.
From drone data and high-resolution satellite imagery to aerial data and IoT sensor data streams—these platforms also offer tools for processing, analysis, and integration.
Real-World Applications and Impact
The integration of ground-based data in remote sensing applications significantly enhances the accuracy and reliability of the results. Here are a few real-world examples:
Vegetation Analysis
In agriculture, remote sensing is used to monitor crop health and predict yields. Ground-based measurements of crop parameters, such as leaf area index, biomass, and chlorophyll content, are essential for calibrating satellite-based vegetation indices.
This calibration ensures that remote sensing models accurately reflect the vegetation or forest conditions on the ground as demonstrated in the figure below. Additionally, tree canopy detection can be easily carried out using platforms like UP42.
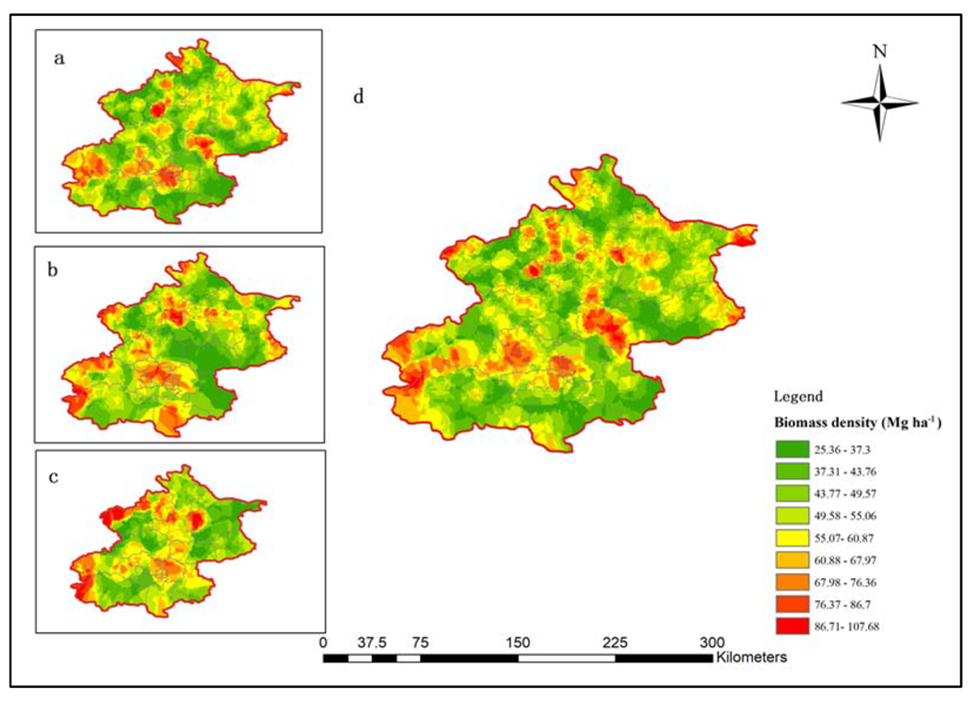
Figure 1: Biomass density distribution in Beijing, China (a) Coniferous forest; (b) Mixed forest; (c) Broadleaf forest; (d) all forest sampling plots.
Water Quality Monitoring
Remote sensing is increasingly being used to assess and monitor water quality in lakes, rivers, and coastal areas.
Ground-based measurements of parameters like turbidity, Secchi Disk Depth (SDD) which is an indicator for water clarity, chlorophyll concentration, and nutrient levels are crucial for calibrating regression models. These models can then predict water quality parameters over large areas, providing essential data for environmental management and policy-making.
For example, satellite-derived estimates of chlorophyll concentration and water clarity in a lake can be validated with in-situ measurements to ensure accurate monitoring of algal blooms, which can be harmful to aquatic life and human health. One such example over Canandaigua Lake is presented in this study and the SDD maps are shown below.
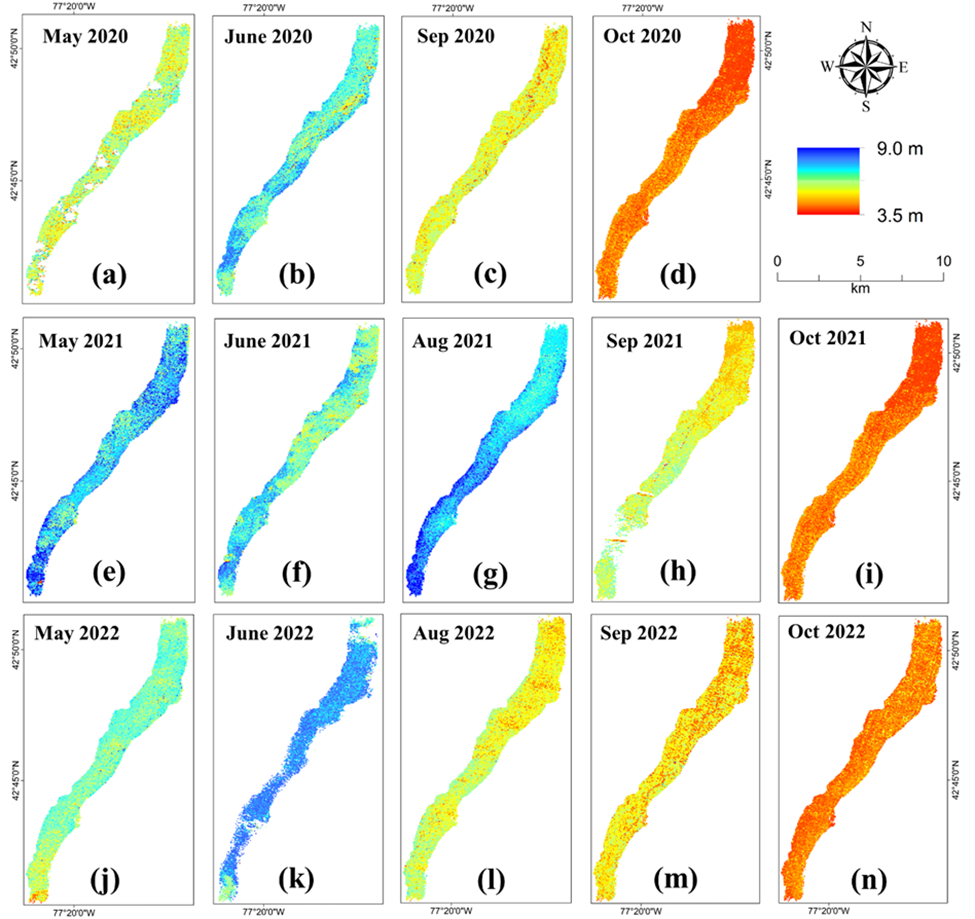
Figure 2: Temporal maps showing the estimated SDD for Canandaigua Lake using one clear imagery for each of the months from June through October for years 2020 (a)–(d), 2021 (e)–(i) and 2022 (j)–(n). The red color indicates the lower values of SDD indicating turbid water and the blue value indicates high values of SDD indicating clearer waters.
Soil Moisture Monitoring
Soil moisture is a critical parameter for agricultural management, drought prediction, and hydrological studies. Remote sensing provides large-scale soil moisture data, but these estimates need to be validated with ground-based measurements as illustrated from a study below.
Ground-based sensors and manual sampling provide accurate soil moisture data that are used to calibrate and validate satellite-derived models. This ensures that the remote sensing models accurately reflect the soil moisture conditions, which is essential for effective water resource management, irrigation planning, and understanding the impacts of climate change on agriculture.
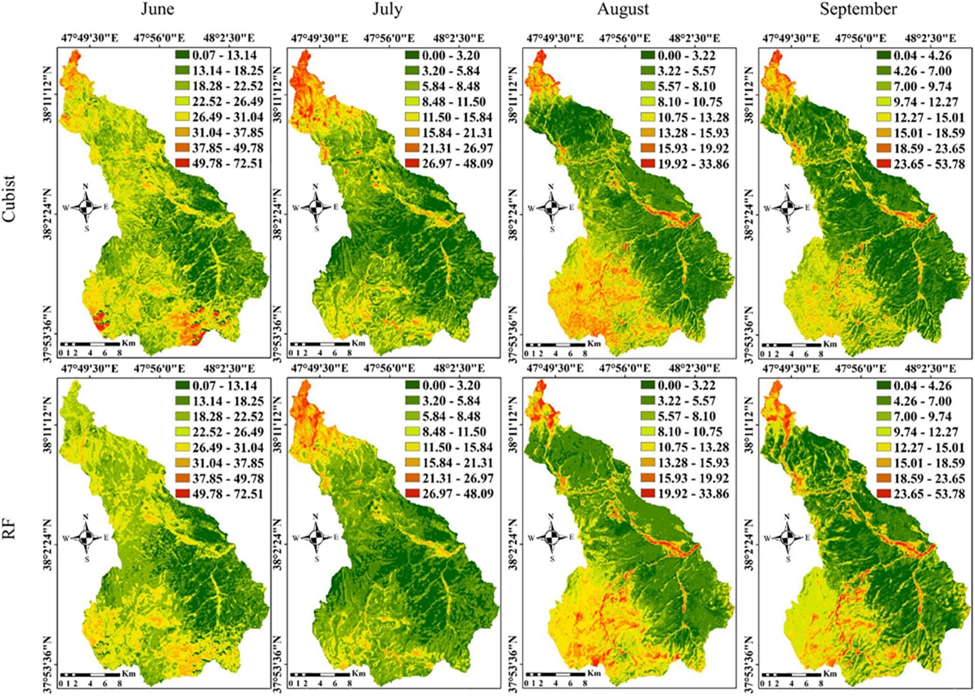
Figure 3: Predictive maps of soil moisture based on the generalized model for both the Cubist and Random Forest MLAs in the study area for the four months.
Conclusion: The Synergy of Ground-Based Data and Remote Sensing
Ground-based data is a cornerstone of ensuring the accuracy and reliability of regression models in remote sensing applications. While remote sensing technology provides a broad overview of environmental conditions, ground-based data offers the detailed, localized information necessary for precise model calibration and validation.
By combining the strengths of remote sensing and ground-based observations, we can achieve more accurate and reliable environmental insights, ultimately leading to better decision-making and resource management. The future of Earth observation and analysis lies in this integrated approach, combining the global perspective of satellites with the detailed, nuanced information provided by ground-based observations.
Emerging technologies like drones, IoT sensors, and AI/ML offer solutions to overcome challenges in ground-based data collection by providing high-resolution data, real-time monitoring, and efficient data processing, reducing costs and enhancing compatibility with satellite data. Therefore, despite the challenges of collecting ground-based data, the integration of new technologies promises to enhance the efficiency and accuracy of this crucial process.
Did you like the article? Read more and subscribe to our monthly newsletter!
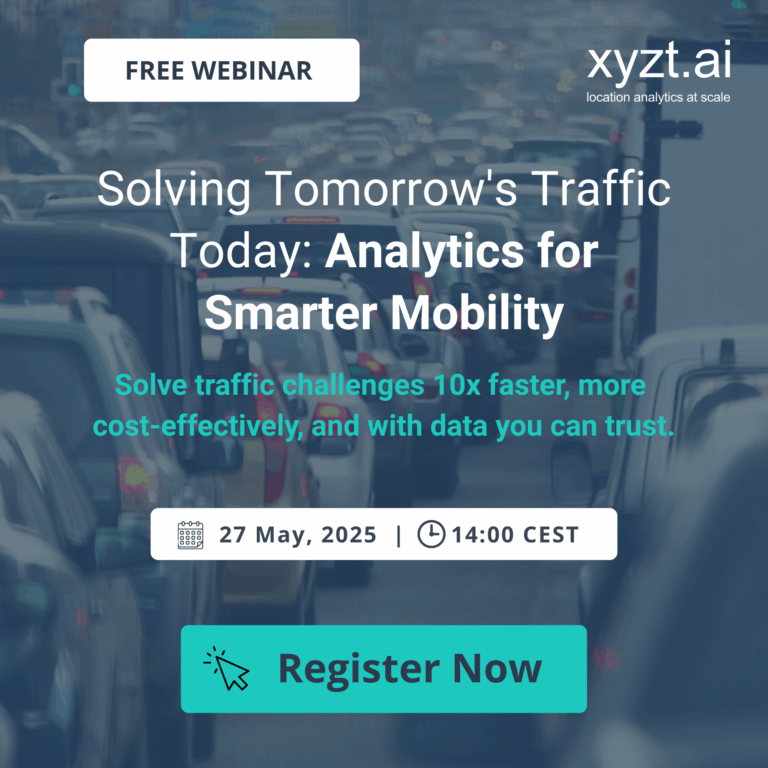
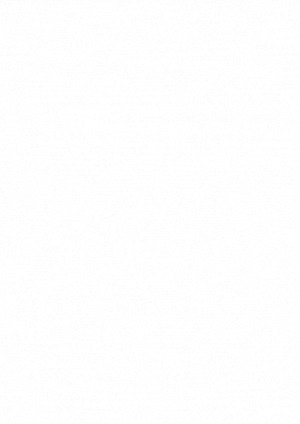