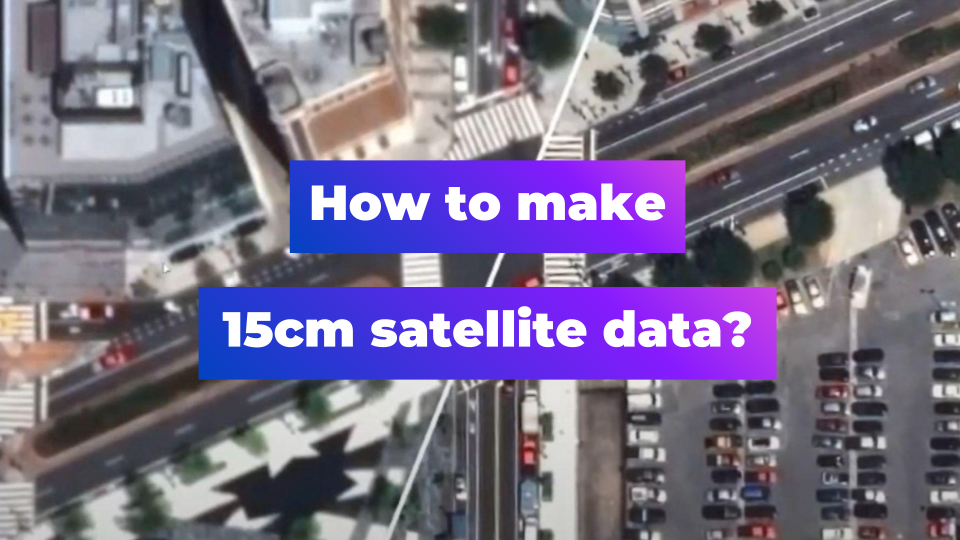
Enhancing Satellite Imagery Readability with Super-resolution Machine Learning Models
The satellite imagery landscape has been utterly transformed in recent years, with a surge in quality and accessibility that has made these images essential for various sectors—from agriculture to urban planning. Yet, even with the highest resolution images available, analysts often grapple with issues in interpretation, wishing for a few pixels more to work with.
AI has at last provided a solution: new super-resolution (SR) models, which could help take satellite imagery to the next level of clarity.
Super-resolution, although not technically enhancing the actual resolution of the data, has the potential to significantly improve the visual quality of satellite imagery, effectively creating High Definition (HD) images. In commercial settings, SR has been employed to refine images from 30cm to 15cm resolution or 50cm to 30cm resolution. Some even ventured to enhance low-resolution open-source data, such as increasing European Sentinel-2 10m data to an impressive 2.5m resolution. What’s more, this enhancement can be applied to all spectral bands.
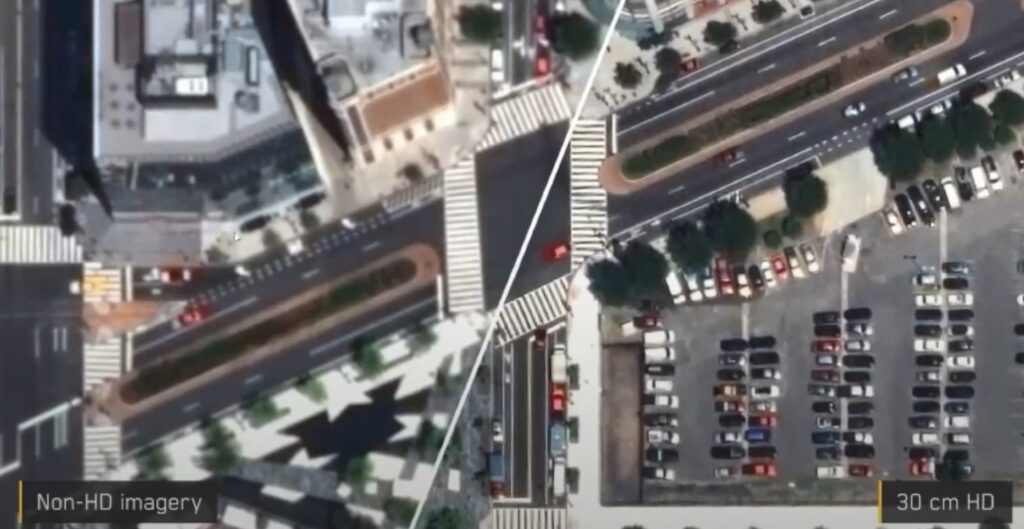
Image from Maxar
Despite its name, super-resolution’s improvements are primarily aesthetic. By generating extra pixels, SR refines the edges of objects and artificially reconstructs details, enhancing the overall visual clarity and readability of the image. However, SR does not unveil any hidden data that wasn’t initially captured. For instance, if an object isn’t present in the original data, SR will not reveal it in the enhanced image. Consequently, while an SR image may contain more pixels than its low-resolution counterpart, the Ground Sampling Distance (GSD) remains unchanged.
Despite these limitations, the advent of super-resolution technology is unlocking numerous new possibilities and practical applications across a wide array of industries. As the technology continues to evolve, its potential to revolutionize satellite imagery and its applications will undoubtedly reach even greater heights.
How does it work, and how is it implemented in satellite imagery?
The concept of super-resolution is not unique to satellite imagery. It is now a fairly widely available machine-learning task used to upscale and improve the details within an image; creating a high resolution output from a low resolution input, usually for aesthetic purposes. By applying an algorithm to the low-resolution image, absent details are filled in, increasing the resolution while maintaining or improving visual quality. Of course, the higher the original input resolution, the better the result in the SR image. SR is particularly good at creating precise edges and enhancing long linear features.
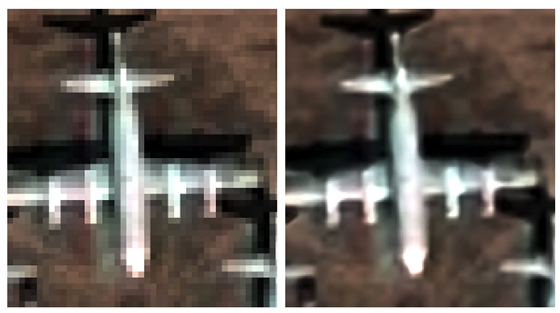
Image from UP42
AI super-resolution models use deep learning techniques, whereby algorithms are trained on large datasets containing pairs of high-resolution and corresponding low-resolution images. This process teaches the model to recognise which data is missing from an image, and therefore how to reconstruct it.
The most common architecture for these AI models is a type of neural network called a convolutional neural network (CNN), which is specifically designed for processing grid-like data—e.g. digital images. Using this method, images are processed through multiple filters, including convolutional and pooling layers, which are able to extract high-level features and textures, thereby enabling the model to recognize complex patterns.
The model is also trained to minimize the difference between the SR output and the true high-resolution image. This is done using a loss function (such as mean squared error or perceptual loss) and encourages the model to produce reconstructed images which are both aesthetically pleasing and accurate.
Additional challenges arise when applying SR to satellite data. Traditional super-resolution algorithms have been developed on RGB images, but to work with satellite imagery they need to be trained on multispectral—or hyperspectral—data, which can have dozens of bands. Atmospheric conditions present additional hurdles to overcome and may be misinterpreted by an AI model.
Notable super-resolution approaches and products
Despite the challenges, there are already several notable products on the market that leverage SR technology, each of which employs cutting-edge machine learning techniques to provide analysts with enhanced detail and readability.
One such example is UP42’s CNN-based SR model, an algorithm which uses multispectral and pan-sharpened image pairs in training (instead of downscaled images). UP42’s model can quadruple the resolution of Pléiades and SPOT imagery, and is available on its marketplace.
Another example is coming from South Korean company Nara Space which developed a super resolution algorithm that improves the readability of Pléiades Neo images from 30 cm to 10 cm. Airbus itself offers Pléiades Neo HD15 product with 15cm enhanced resolution.
Maxar’s HD technology has also had significant success, producing 15cm SR images which consistently rate at 6–7 on the National Imagery Interpretability Scale (NIIS). (The NIIS is a subjective scale used by image analysts to rank the quality of aerial imagery from 0–9, where, for example, basic land use is intelligible in 1, and a car licence plate can be read at 8).
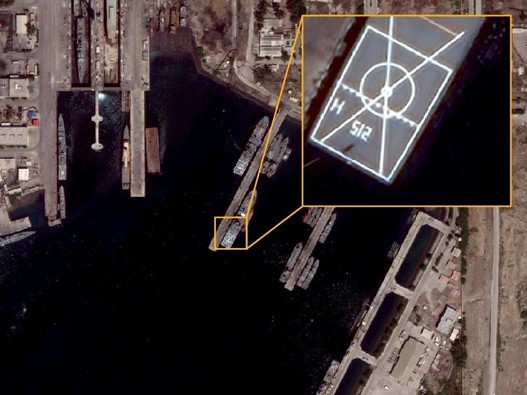
Image from Maxar
Benefits and applications of super-resolution models in satellite imagery
SR models in satellite imagery offer opportunities across a multitude of applications, including mapping, monitoring, feature identification and analytics. By enhancing the visual experience and reducing pixelation, these models provide analysts with actionable information, allowing them to discern smaller features on the ground, and thereby contribute to better decision-making.
In urban planning, SR enables more precise identification of features, and reduces error rates dramatically in identifying smaller objects like lampposts, solar panels, road signs and vehicles. Enhanced images of infrastructure conditions may prove advantageous for asset monitoring and disaster management. SR models not only help with counting features, but can also enhance the textural information of vegetation and tree canopies, which has applications for agriculture and climate monitoring.
It’s also worth mentioning that SR has benefits for both new and archive imagery. For a start, it can help to increase the global supply of 30cm imagery by improving low-resolution archive data to match newly-collected 30cm native data. Tasking the highest-resolution satellites, meanwhile, is often prohibitively expensive, and SR models can provide a cost-effective alternative for a wide range of applications. In fact, the readability of satellite data when enhanced to 15cm becomes very close to 10cm aerial data. In some use cases, SR satellite imagery data could replace aerial data—at a fraction of the cost.
One particularly significant advantage of SR models is their ability to improve the accuracy of other AI models applied to satellite data. For example, early tests by Maxar showed that machine-learning models detecting cars had their accuracy improved by 15-40% when working with images enhanced by SR. In some cases, SR models even have improved interpretability over native 30cm data—particularly with regard to linear features and reconstructing markings on the ground or on vehicles.
Super-resolution: overcoming challenges to provide more reliable geospatial data
While super-resolution models offer a range of advantages, implementing them in satellite imagery is not without challenges. Aside from the primary concern of the model’s accuracy, other technical limitations can also pose significant challenges, as applying a SR machine learning model to satellite data can require a huge amount of processing power and data storage. Ethical and legal considerations, including privacy concerns and data usage restrictions, must also be addressed, while integrating super-resolution models with existing workflows and systems can prove complex and time-consuming. All of these challenges could potentially impede the adoption of SR in certain applications; however, with the major satellite companies like Maxar and marketplaces like UP42 all striving to overcome them, super-resolution satellite imagery is becoming a reality.
Super-resolution machine learning models offer an innovative solution to enhance satellite imagery readability for both human analysts and AIs, providing higher quality data for various applications. Their potential benefits in terms of improved decision-making and AI performance make them a hugely valuable addition to the analyst’s toolbox—and potentially a cost-effective alternative to native 30cm, or even 10cm aerial data, for many customers. As technology continues to advance, we can expect further improvements in super-resolution techniques and their applications in satellite imagery, paving the way for even more reliable geospatial data.
Did you like the blog post? Read more and subscribe to our monthly newsletter!
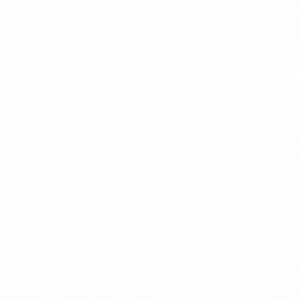