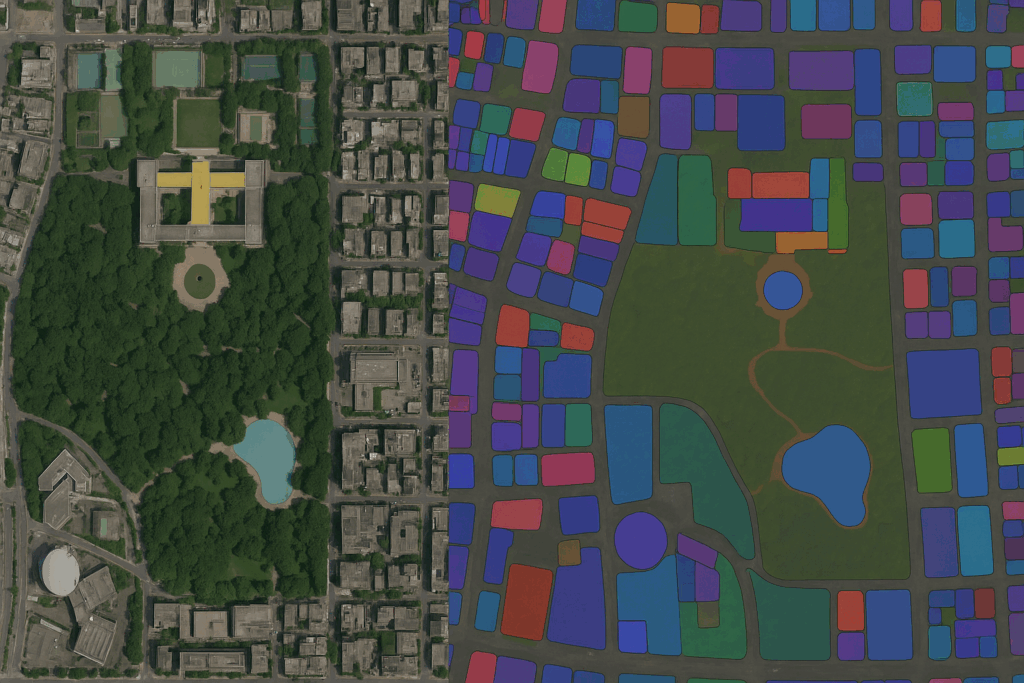
AI Foundation Models for Geospatial and Earth observation: A New Era of Earth Understanding
Foundation models, which are large, pretrained AI systems, have had a significant impact on natural language processing, computer vision, and now, Earth observation. These models are trained on extensive, diverse, and frequently multimodal datasets, enabling them to generalize effectively across various tasks, regions, and sensors. In the geospatial domain, they enable capabilities to monitor, analyze, and predict changes on our planet.
But how are foundation models different from LLMs (Large Language Models)?
Large Language Models (LLMs) are a specific type of foundation model designed primarily for tasks involving natural language. While all LLMs are foundation models, not all foundation models are LLMs. Foundation models can span various domains—including vision, language, audio, or even multimodal tasks—whereas LLMs focus specifically on understanding and generating human language. The table below summarizes the key differences and relationship between them:
Recent advancements signal a new wave of geospatial intelligence that leverages foundation models to address challenges in climate change, disaster response, urbanization, and agriculture. This article explores the impact of foundation models on geospatial analysis.
What Are Foundation Models in the Geospatial Context?
Foundation models are deep learning architectures trained on extensive datasets to learn general representations that can be fine-tuned for specific tasks. In EO, they are often multimodal, having been trained on satellite imagery, radar data, topography, weather, and even text-based reports. This allows them to perform a wide array of geospatial tasks without being retrained from scratch.
Unlike traditional EO algorithms such as NDVI, which are handcrafted indices designed to measure specific phenomena (e.g., vegetation health using spectral bands), foundation models automatically learn complex patterns and features from data. They do not rely on fixed rules or predefined formulas but instead extract hierarchical representations through large-scale learning. This makes them more flexible and powerful when addressing diverse applications like land use mapping, deforestation tracking, or flood detection. Rather than building a separate model for each task, a foundation model can be adapted to multiple domains, improving efficiency and performance at scale.
Key Research and Industry Examples
1. IBM’s TerraMind
IBM’s recently open-sourced TerraMind project, in collaboration with the European Space Agency (ESA), is a state-of-the-art foundation model for Earth observation. It integrates nine modalities – distinct types of data such as optical imagery, SAR (synthetic aperture radar), elevation models, weather data, and textual annotations.
Trained on over 524 million EO tiles, TerraMind is capable of performing semantic segmentation (e.g., detecting water bodies, vegetation types), classification (e.g., urban vs. rural), and even inferring missing modalities. Its performance significantly surpasses that of traditional single-task models, particularly in regions with limited data resources.
2. Google’s Geospatial Reasoning Models
In its April 2025 blog post, Google introduced models that integrate EO data with language and contextual data to provide geospatial reasoning. These models are used in:
- Flood forecasting
- Real-time wildfire mapping
- Population displacement tracking
By integrating satellite data with topography, hydrology, and language inputs such as field reports or emergency calls, the models can reason beyond pixels to understand context and risk.
3. Meta’s Segment Anything Model (SAM) in Remote Sensing
Although not exclusively geospatial, SAM has been fine-tuned by researchers to segment satellite images – e.g., identifying buildings, agricultural fields, or glaciers. This “click-and-segment” approach democratizes EO data interpretation for non-experts.
Other Surveys and Research
- Foundation Models for Generalist Geospatial Artificial Intelligence:
- Introduces a framework for pre-training and fine-tuning foundation models on extensive geospatial data, demonstrating applications in cloud gap imputation and flood mapping.
- arXiv Preprint
- Vision Foundation Models in Remote Sensing: A Survey:
- Provides a comprehensive overview of vision foundation models in remote sensing, discussing architectures, datasets, and methodologies.
- arXiv Preprint
- Foundation Models for Remote Sensing and Earth observation: A Survey:
- Systematically reviews remote sensing foundation models, categorizing them into visual foundation models, vision-language models, and large language models, and discusses their applications and challenges.
- arXiv Preprint
Applications Across Domains
Domain | Example Use Case | Description |
Earth Observation | Clay Foundation Model by DevelopmentSeed | This foundation model is versatile and open-source, making it suitable for a variety of Earth observation applications. It is capable of handling diverse data sources, including satellite, drone, and SAR imagery. It employs a two-stage transformer architecture and a flexible data pipeline for scalable training. |
Geospatial AI | Vision-Language Queryable Earth by Element84 | It utilizes vision-language models like SkyCLIP and RemoteCLIP to enable text-based geospatial queries, allowing users to retrieve satellite images based on natural language descriptions. |
Geospatial AI | Natural Language Geocoding by Element84 | It employs natural language processing to interpret user queries (e.g., “Show me algal blooms within 2 miles of Cape Cod”) and displays relevant geospatial data, enhancing accessibility for non-experts. |
Geospatial AI | Cloud Detection in Satellite Imagery by Element84 | It develops machine learning models to automatically detect and remove clouds from satellite images, improving the accuracy of downstream geospatial analyses. |
Agriculture | Crop health prediction | Foundation models detect drought stress or disease outbreaks using multispectral imagery. |
Disaster Response | Post-earthquake damage assessment | Models segment damaged buildings and blocked roads using SAR + optical data. |
Climate Monitoring | Glacier retreat tracking | Foundation models segment ice cover over time to monitor melting rates. |
Urban Planning | Informal settlement mapping | Combining EO with census or text data to detect unplanned urban sprawl. |
Forestry | Illegal logging detection | Models detect canopy loss in near real-time across large forested areas. |
Future Directions
What’s Next? The future of this field is bright, and open-source software is an integral part of it. The development of open-source foundation models is expected to accelerate, enabling academic institutions and government agencies to leverage advanced AI capabilities without incurring substantial computational costs. Domain-specific transformer architectures will be fine-tuned for Earth observation time-series analysis and multimodal geospatial data fusion. Deployment at the edge – on drones and mobile devices – will support real-time, in-field decision-making for applications such as precision agriculture and wildfire monitoring. Additionally, integration with policy frameworks will allow these models to directly contribute to tracking progress on the United Nations Sustainable Development Goals (SDGs), including indicators such as access to clean water and land degradation.
Conclusion
Foundation models in EO represent a leap toward general-purpose geospatial intelligence. By learning from diverse data across the globe, they enable more accurate, scalable, and context-aware analysis of our dynamic Earth. As these models become more open, interpretable, and efficient, they will transform how we respond to environmental challenges and opportunities across science, policy, and society.
Did you like the article? Read more and subscribe to our monthly newsletter!